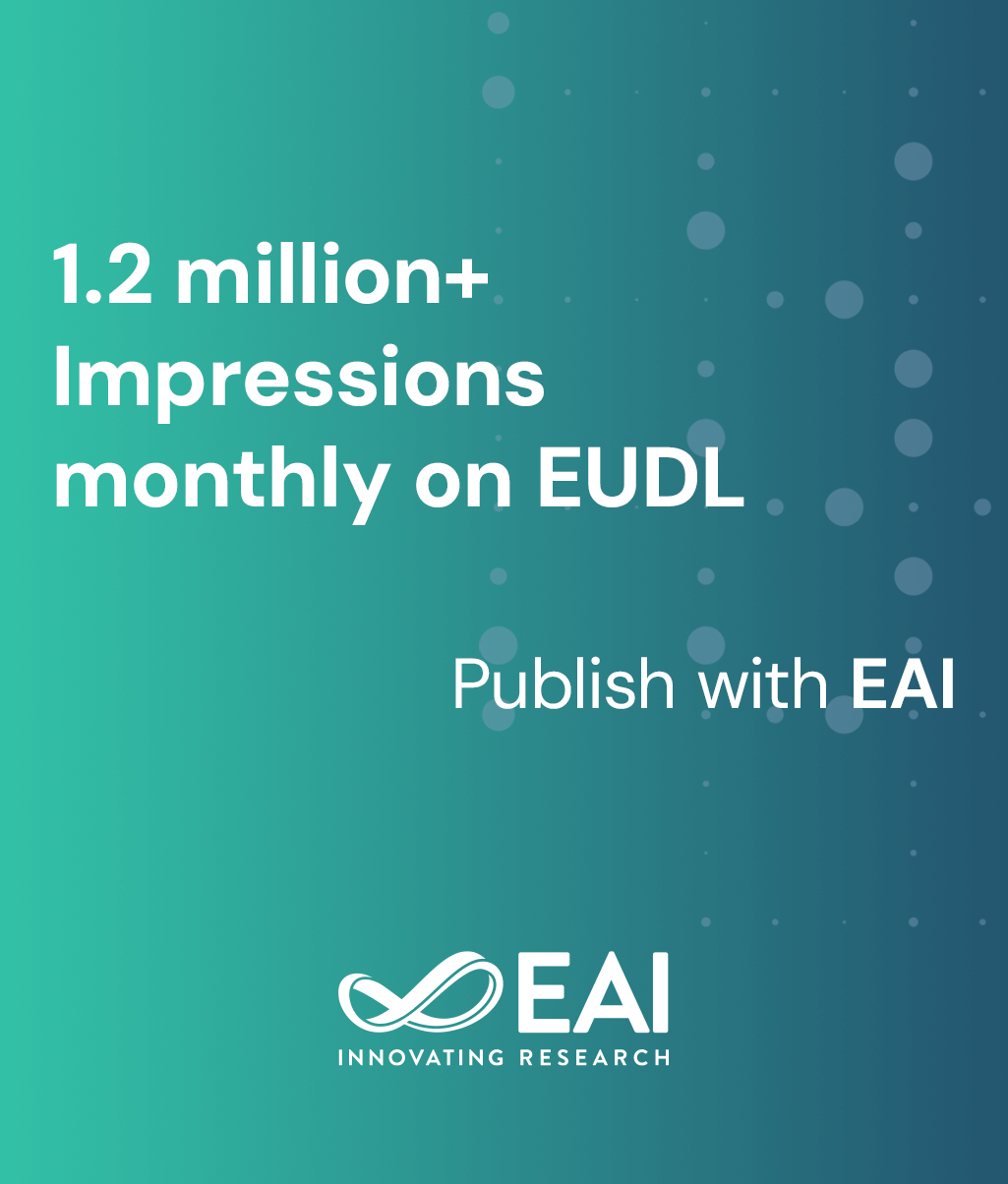
Research Article
Combining Human and Machine Computing Elements for Analysis via Crowdsourcing
@INPROCEEDINGS{10.4108/icst.collaboratecom.2014.257298, author={Brian Blake and Julian Jarrett and Iman Saleh and Rohan Malcolm and Sean Thorpe and Tyrone Grandison}, title={Combining Human and Machine Computing Elements for Analysis via Crowdsourcing}, proceedings={10th IEEE International Conference on Collaborative Computing: Networking, Applications and Worksharing}, publisher={IEEE}, proceedings_a={COLLABORATECOM}, year={2014}, month={11}, keywords={crowdsouring; experimentation; elastic systems}, doi={10.4108/icst.collaboratecom.2014.257298} }
- Brian Blake
Julian Jarrett
Iman Saleh
Rohan Malcolm
Sean Thorpe
Tyrone Grandison
Year: 2014
Combining Human and Machine Computing Elements for Analysis via Crowdsourcing
COLLABORATECOM
IEEE
DOI: 10.4108/icst.collaboratecom.2014.257298
Abstract
Crowd computing leverages human input in order to execute tasks that are computationally expensive, due to complexity and/or scale. Combined with automation, crowd computing can help solve problems efficiently and effectively. In this work, we introduce an elasticity framework that adaptively optimizes the use of human and automated software resources in order to maximize overall performance. This framework includes a quantitative model that supports elasticity when performing complex tasks. Our model defines a task complexity index and an elasticity index that is used to aid in decision support for assigning tasks to respective computing elements. Experiments demonstrate that the framework can effectively optimize the use of human and machine computing elements simultaneously. Also, as a consequence, overall performance is significantly enhanced.