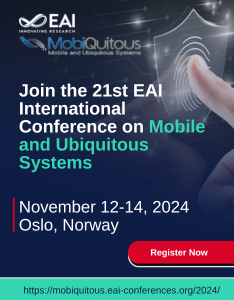
Research Article
Piecewise Linear Dynamical Model for Actions Clustering from Inertial Body Sensors with Considerations of Human Factors
@INPROCEEDINGS{10.4108/icst.bodynets.2014.257043, author={Jiaqi Gong and Philip Asare and John Lach and Yanjun Qi}, title={Piecewise Linear Dynamical Model for Actions Clustering from Inertial Body Sensors with Considerations of Human Factors}, proceedings={9th International Conference on Body Area Networks}, publisher={ICST}, proceedings_a={BODYNETS}, year={2014}, month={11}, keywords={time series clustering human motion analysis inertial body sensor networks linear dynamical system stimulus detection}, doi={10.4108/icst.bodynets.2014.257043} }
- Jiaqi Gong
Philip Asare
John Lach
Yanjun Qi
Year: 2014
Piecewise Linear Dynamical Model for Actions Clustering from Inertial Body Sensors with Considerations of Human Factors
BODYNETS
ACM
DOI: 10.4108/icst.bodynets.2014.257043
Abstract
The efficacy of many Body Sensor Network (BSN) applications relies on the accurate temporal clustering of human motion into actions on various time scales. This is typically done with the use of inertial sensors and processing algorithms that try to extract such information from the sensor data. Two human factors in real-world deployments make such information extraction challenging: mounting errors (where sensor displacement and orientation do not match what is assumed by processing algorithms) and insecure mounting (where sensors are loosely worn causing them to shake during operations). In order to enhance the robustness of human actions clutsering from real-world BSN data, this work leverages dynamical systems modeling with the considerations of human factors. By proposing a computational body-model framework called the piecewise linear dynamical model (PLDM), we derive a robust method to segment time series data of inertial BSNs in real-world deployment with human factors into motion primitives and actions. We test the proposed method on two different inertial BSNs datasets and extract actions on different temporal scales. The experimental results demonstrate the effectiveness of our approach.