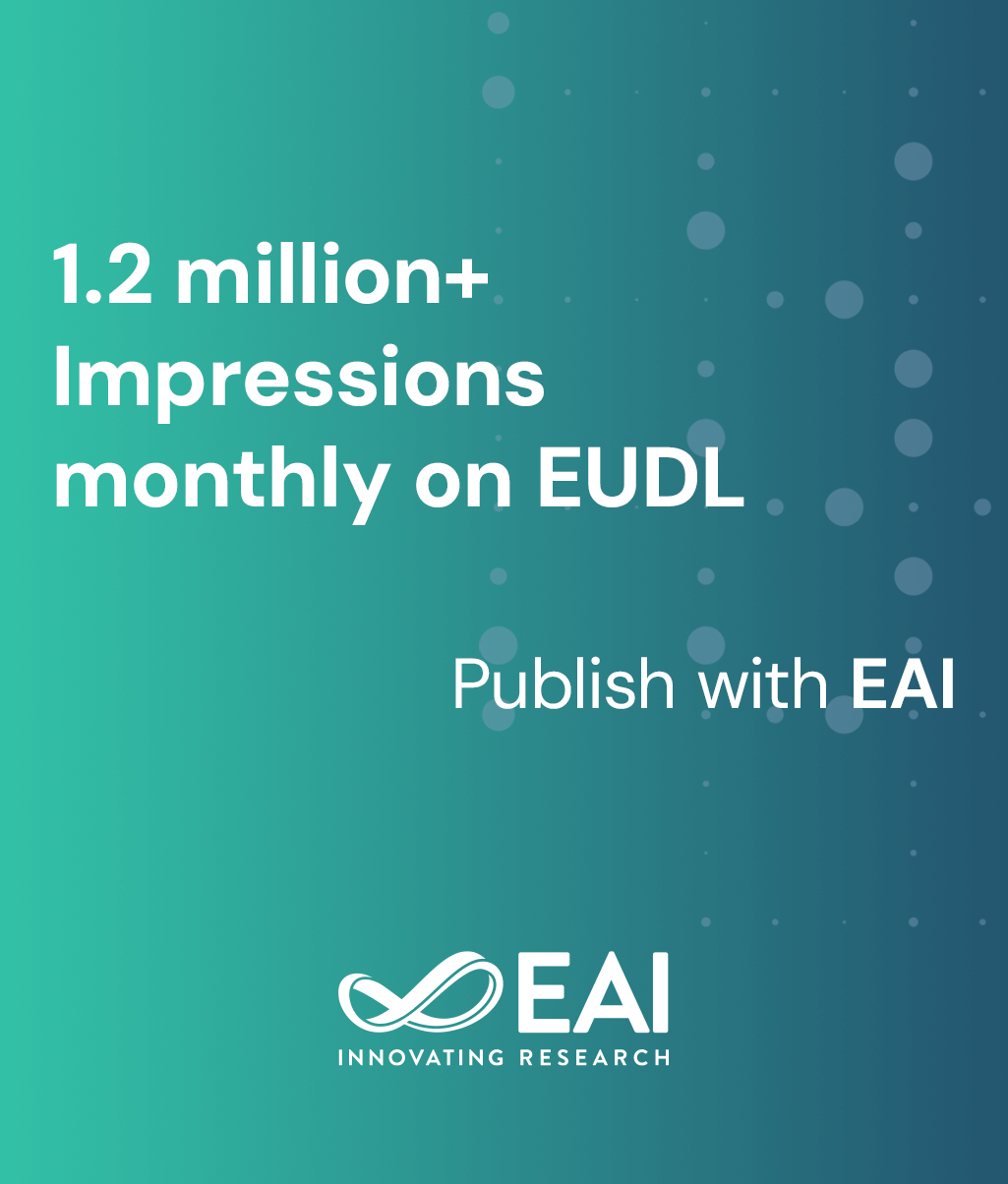
Research Article
Detections of IoT Attacks via Machine Learning-Based Approaches with Cooja
@ARTICLE{10.4108/eetiot.v7i28.324, author={Ali Hamid Farea and Kerem K\'{y}\`{e}\'{y}k}, title={Detections of IoT Attacks via Machine Learning-Based Approaches with Cooja}, journal={EAI Endorsed Transactions on Internet of Things}, volume={7}, number={28}, publisher={EAI}, journal_a={IOT}, year={2022}, month={4}, keywords={IoT security, Attacks, Machine Learning-based approaches, Decision tree-based models, Cooja simulator}, doi={10.4108/eetiot.v7i28.324} }
- Ali Hamid Farea
Kerem Küçük
Year: 2022
Detections of IoT Attacks via Machine Learning-Based Approaches with Cooja
IOT
EAI
DOI: 10.4108/eetiot.v7i28.324
Abstract
Once hardware becomes "intelligent", it is vulnerable to threats. Therefore, IoT ecosystems are susceptible to a variety of attacks and are considered challenging due to heterogeneity and dynamic ecosystem. In this study, we proposed a method for detecting IoT attacks that are based on ML-based approaches that release the final decision to detect IoT attacks. However, we have implemented three attacks as a sample in the IoT via Contiki OS to generate a real dataset of IoT-based features containing a mix of data from malicious nodes and normal nodes in the IoT network to be utilized in the ML-based models. As a result, the multiclass random decision forest ML-based model achieved 98.9% overall accuracy in detecting IoT attacks for the real novel dataset compared to the decision tree jungle, decision forest tree regression, and boosted decision tree regression, which achieved 87.7%, 93.2%, and 87.1%, respectively. Thus, the decision tree-based approach efficiently manipulates and analyzes the KoÜ-6LoWPAN-IoT dataset, generated via the Cooja simulator, to detect inconsistent behavior and classify malicious activities.
Copyright © 2022 Ali Hamid Farea et al., licensed to EAI. This is an open access article distributed under the terms of the Creative Commons Attribution license, which permits unlimited use, distribution, and reproduction in any medium so long as the original work is properly cited.