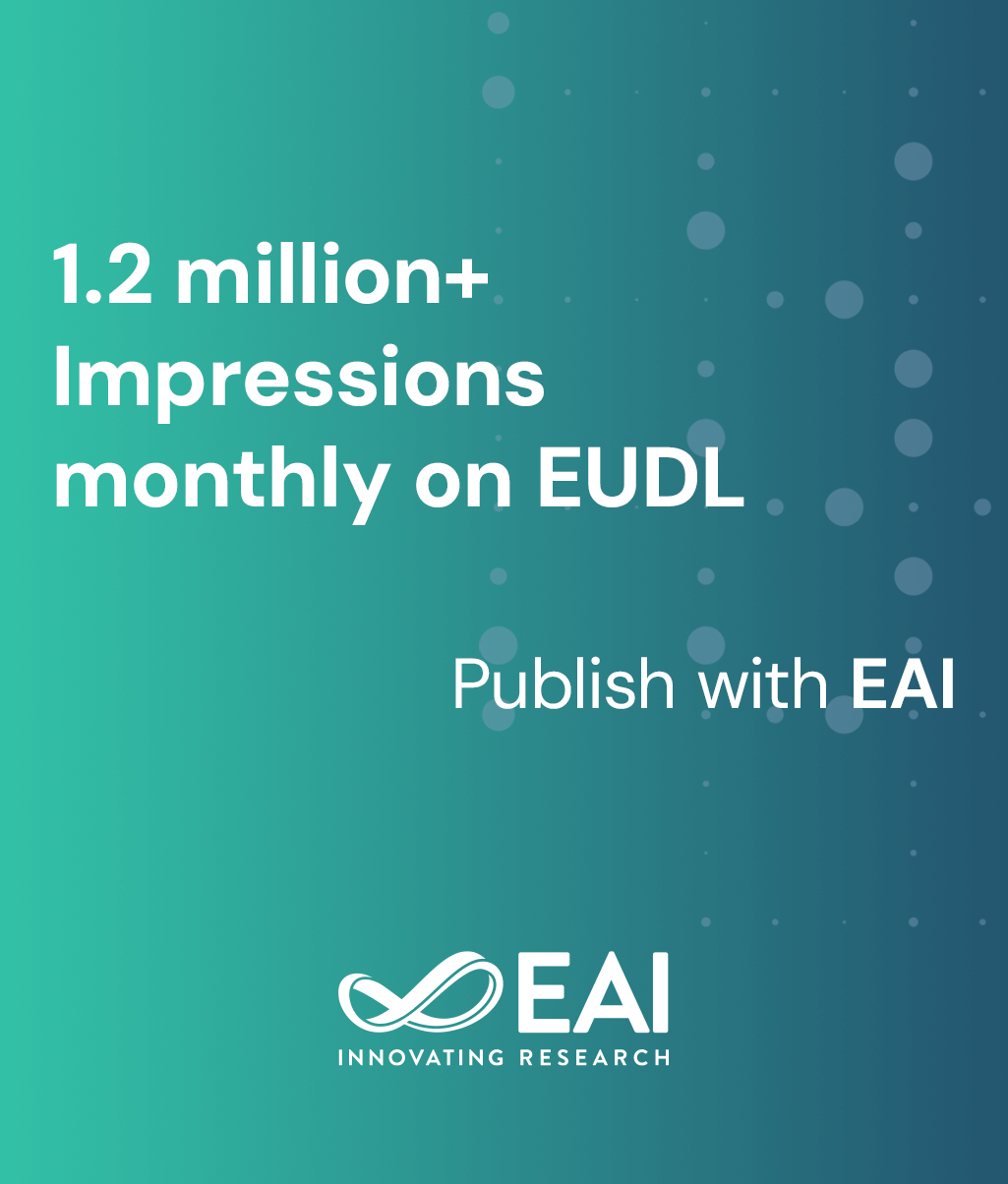
Research Article
Research on Credit Card Overdue Risk Prediction based on CatBoost Model
@INPROCEEDINGS{10.4108/eai.8-12-2023.2344364, author={Zhihui Zhuo}, title={Research on Credit Card Overdue Risk Prediction based on CatBoost Model}, proceedings={Proceedings of the 5th Management Science Informatization and Economic Innovation Development Conference, MSIEID 2023, December 8--10, 2023, Guangzhou, China}, publisher={EAI}, proceedings_a={MSIEID}, year={2024}, month={4}, keywords={credit card; expected risk; catboost model; optuna; risk prediction}, doi={10.4108/eai.8-12-2023.2344364} }
- Zhihui Zhuo
Year: 2024
Research on Credit Card Overdue Risk Prediction based on CatBoost Model
MSIEID
EAI
DOI: 10.4108/eai.8-12-2023.2344364
Abstract
At present, credit card business has become an indispensable financial service for commercial banks and individuals. With the development of credit card business in contemporary transcations, the overdue risk has extremely influenced the utilization of credit cards and affect the economical environment. Existing machine learning models have achieved promising performance toward complex data and become one of most widely used prediction method especifically based on Optuna optimization, which provides valuable insights for improving the level of credit card risk control in commercial banks. In this work, we initially explores transaction flow data that best reflects customer behavior and conducts in-depth analysis of credit card delinquency-related behaviors using theories including behavioral economics and information asymmetry. Furthermore, we construct a CatBoost model based on Optuna hyperparameter optimization to predict credit card delinquency risk. The experiment collects credit card transaction data from various commercial banks and conducts further predictive analysis, providing improvement recommendations that can be referenced for credit card risk management in banks. From our extensive simulations, we can conclude that our model can achieve acceptable prediction accuracy with reasonable responsing costs.