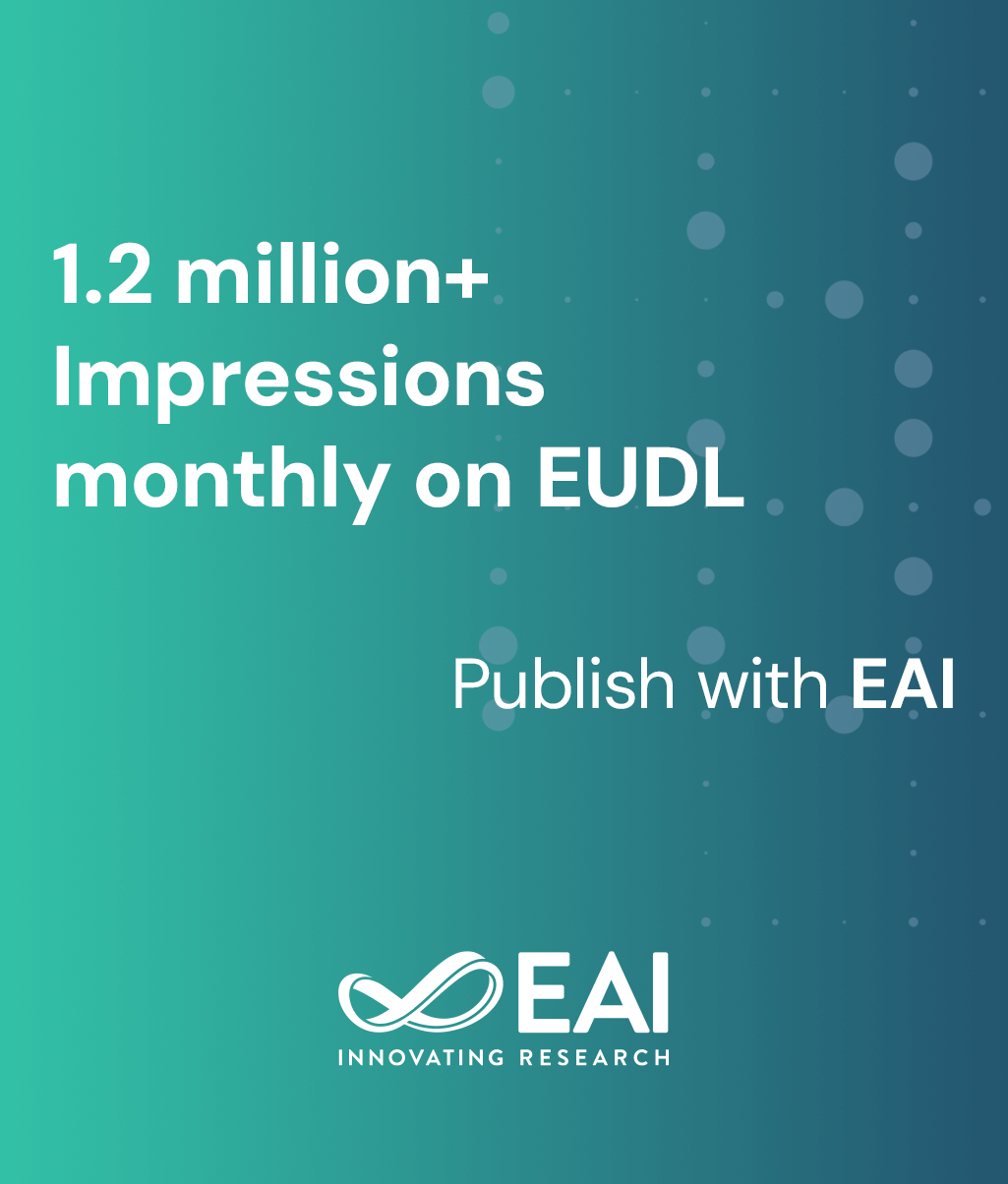
Research Article
Machine Learning and Predictive Analysis of Fossil Fuels Consumption in Mid-Term
@ARTICLE{10.4108/eai.28-12-2017.153522, author={Mahmood Amerion and Mohammadmehdi Hosseini and Abdorreza Alavi Gharahbagh and Mohsen Amerion}, title={Machine Learning and Predictive Analysis of Fossil Fuels Consumption in Mid-Term}, journal={EAI Endorsed Transactions on Scalable Information Systems}, volume={4}, number={15}, publisher={EAI}, journal_a={SIS}, year={2018}, month={1}, keywords={Parallel Processing, Information Systems, Scalability}, doi={10.4108/eai.28-12-2017.153522} }
- Mahmood Amerion
Mohammadmehdi Hosseini
Abdorreza Alavi Gharahbagh
Mohsen Amerion
Year: 2018
Machine Learning and Predictive Analysis of Fossil Fuels Consumption in Mid-Term
SIS
EAI
DOI: 10.4108/eai.28-12-2017.153522
Abstract
In economies that are dependent on fossil fuel revenues, Realization of long-term plans, mid-term and annual budgeting requires a fairly accurate estimation of the amount of consumption and its price fluctuations. Accordingly, the present study is using machine learning techniques to predict the usage of fossil fuels (Diesel, Black oil, Heating oil, and Petrol) in mid-term. Exponential Smoothing, a model of time series and the Neural Network model have been applied on the actual usage data obtained from Shahroud area from 2010 to 2015. For estimation of predictive value by Neural Network method, the training and testing samples, the highest and lowest errors with a range of 41% -0.89% and 88% -3% for the Mean Absolute Percent Deviation are the most appropriate predictions for Petrol consumption. And in the Single Exponential Smoothing, the forecast rate for each product is estimated on a quarterly as well as monthly basis.
Copyright © 2017 Mahmood Amerion et al., licensed to EAI. This is an open access article distributed under the terms of the Creative Commons Attribution licence (http://creativecommons.org/licenses/by/3.0/), which permits unlimited use, distribution and reproduction in any medium so long as the original work is properly cited.