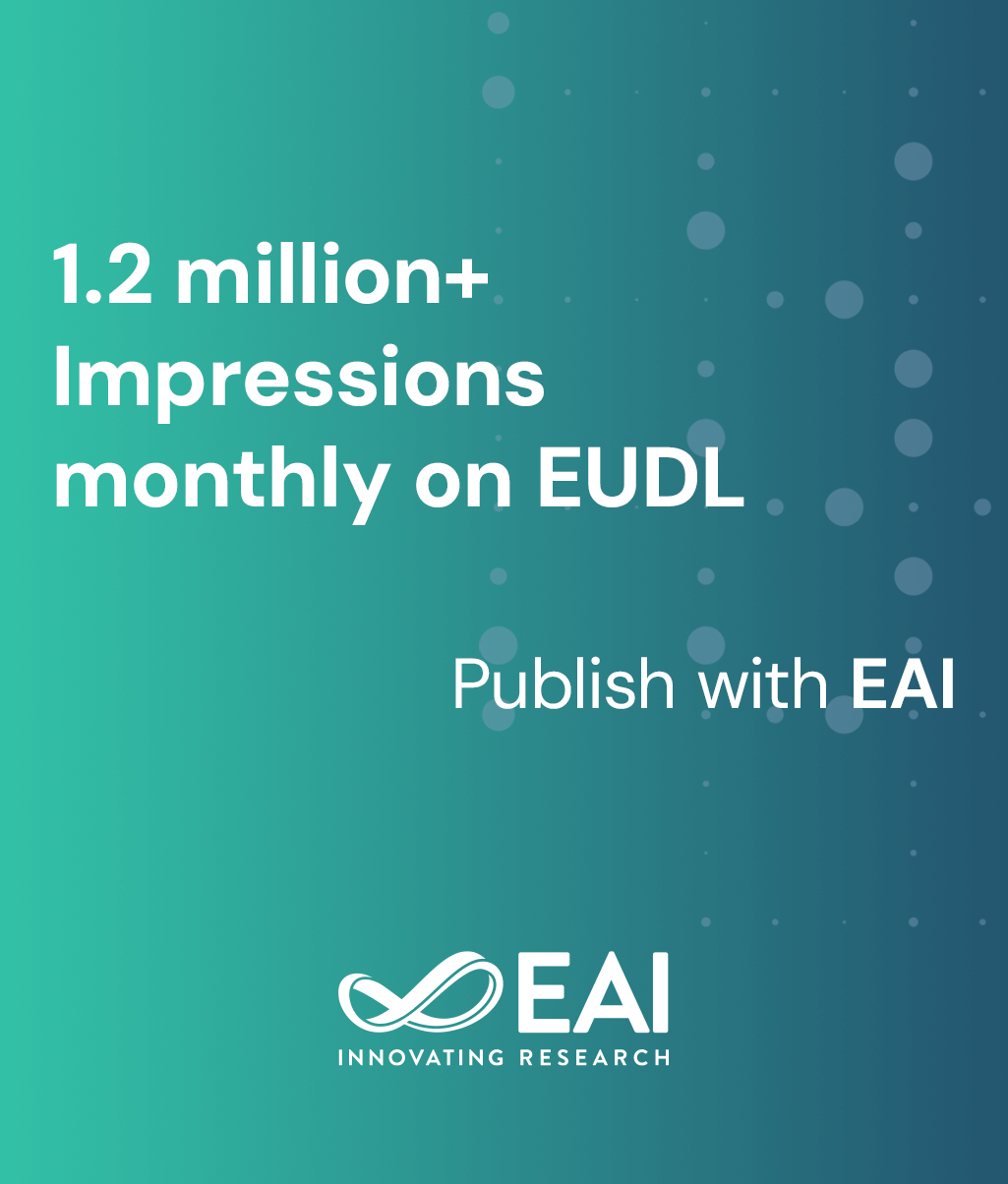
Research Article
A Comparative Analysis of Feature Extraction Methods for Classifying Colon Cancer Microarray Data
@ARTICLE{10.4108/eai.25-9-2017.153147, author={M.O. Arowolo and R.M. Isiaka and S.O. Abdulsalam and Y.K. Saheed and K.A. Gbolagade}, title={A Comparative Analysis of Feature Extraction Methods for Classifying Colon Cancer Microarray Data}, journal={EAI Endorsed Transactions on Scalable Information Systems}, volume={4}, number={14}, publisher={EAI}, journal_a={SIS}, year={2017}, month={9}, keywords={Dimensionality Reduction, Principal Component Analysis, Partial Least Square, Support Vector Machine.}, doi={10.4108/eai.25-9-2017.153147} }
- M.O. Arowolo
R.M. Isiaka
S.O. Abdulsalam
Y.K. Saheed
K.A. Gbolagade
Year: 2017
A Comparative Analysis of Feature Extraction Methods for Classifying Colon Cancer Microarray Data
SIS
EAI
DOI: 10.4108/eai.25-9-2017.153147
Abstract
Feature extraction is a proficient method for reducing dimensions in the analysis and prediction of cancer classification. Microarray procedure has shown great importance in fetching informative genes th at needs enhancement in diagnosis. Microarray data is a challenging task due to high dimensional-low sample dataset with a lot of noisy or irrelevant genes and missing data. In this paper, a comparative study to demonstrate the effectiveness of feature ext raction as a dimensionality reduction process is proposed, and concludes by investigating the most efficient approach that can be used to enhance classification of microarray. Principal Component Analysis (PCA) as an unsupervised technique and Partial Least Square (PLS) as a supervised technique are considered, Support Vector Machine (SVM) classifier were applied on the dataset. The overall result shows that PLS algorithm provides an improved performance of about 95.2% accu racy compared to PCA algorithms.
Copyright © 2017 M.O Arowolo et al., licensed to EAI. This is an open access article distributed under the terms of the Creative Commons Attribution licence (http://creativecommons.org/licenses/by/3.0/), which permits unlimited use, distribution and reproduction in any medium so long as the original work is properly cited.