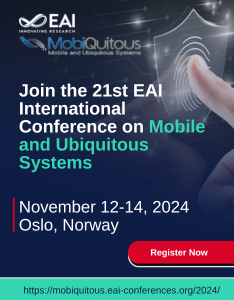
Research Article
Wi-Fi Hotspot Auto-Discovery: A Practical & Energy-Aware System for Smart Objects using Cellular Signals
@ARTICLE{10.4108/eai.22-7-2015.2260071, author={Nithyananthan Poosamani and Injong Rhee}, title={Wi-Fi Hotspot Auto-Discovery: A Practical \& Energy-Aware System for Smart Objects using Cellular Signals}, journal={EAI Endorsed Transactions on Energy Web}, volume={2}, number={5}, publisher={EAI}, journal_a={EW}, year={2015}, month={8}, keywords={wi-fi sensing, cellular signals, smart objects, location fingerprinting, energy-efficiency}, doi={10.4108/eai.22-7-2015.2260071} }
- Nithyananthan Poosamani
Injong Rhee
Year: 2015
Wi-Fi Hotspot Auto-Discovery: A Practical & Energy-Aware System for Smart Objects using Cellular Signals
EW
EAI
DOI: 10.4108/eai.22-7-2015.2260071
Abstract
The Internet of Things (IoT) paradigm aims to interconnect a variety of heterogeneous Smart Objects (SO) using energy-efficient methodologies and standard communication protocols. A majority of consumer devices sold today come equipped with wireless LAN and cellular technology to connect with the world-wide network. To discover Wi-Fi hot spots, there is a need for constant scanning of Wi-Fi radio in these devices and results in significant battery drain. We present PRiSM, a practical system to automatically locate Wi-Fi hotspots while Wi-Fi radio is turned off, by using the statistical characteristics of cellular signals. Cellular signals are received at zero extra cost in mobile devices and hence PRiSM is highly energy-efficient. It is a lightweight client-side only implementation and needs no prior knowledge on floor plans or wireless infrastructure. We implement PRiSM on Android-based devices and show up to 96% of energy savings in Wi-Fi sensing operations which is equivalent to saving up to 16% of total battery capacity, together with an average prediction accuracy of up to 98%.
Copyright © 2015 N. Poosamani and I. Rhee, licensed to EAI. This is an open access article distributed under the terms of the Creative Commons Attribution licence (http://creativecommons.org/licenses/by/3.0/), which permits unlimited use, distribution and reproduction in any medium so long as the original work is properly cited.