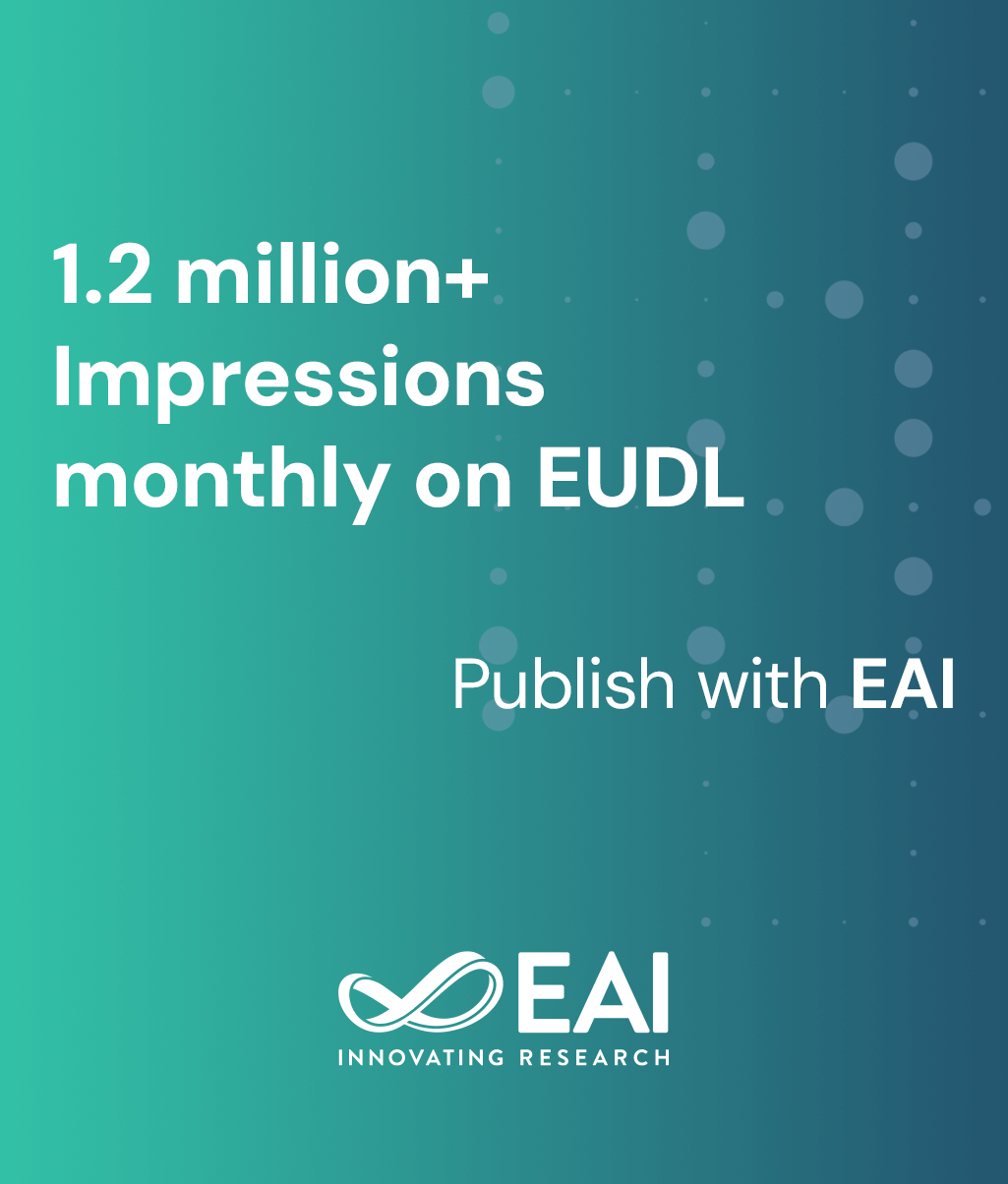
Research Article
A Combination of Off-line and On-line Learning to Classifier Grids for Object Detection
@ARTICLE{10.4108/eai.2-5-2016.151208, author={Nguyen Dang Binh}, title={A Combination of Off-line and On-line Learning to Classifier Grids for Object Detection}, journal={EAI Endorsed Transactions on Context-aware Systems and Applications}, volume={3}, number={9}, publisher={EAI}, journal_a={CASA}, year={2016}, month={5}, keywords={context-based learning, classifier grids, object detection, online learning.}, doi={10.4108/eai.2-5-2016.151208} }
- Nguyen Dang Binh
Year: 2016
A Combination of Off-line and On-line Learning to Classifier Grids for Object Detection
CASA
EAI
DOI: 10.4108/eai.2-5-2016.151208
Abstract
We propose a new method for object detection by combining off-line and on-line boosting learning to classifier grids based on visual information without human intervention concerned to intelligent surveillance system. It allows for combine information labeled and unlabeled use different contexts to update the system, which is not available at off-line training time. The main goal is to develop an adaptive but robust system and to combine prior knowledge with new information in an unsupervised learning framework that is learning 24 hours a day and 7 days a week. We use co-training strategy by combining off-line and on-line learning to the classifier grids. The proposed method is practically favorable as it meets the requirements of real-time performance, accuracy and robustness. It works well with reasonable amount of training samples and is computational efficiency. Experiments on detection of objects in challenging data sets show the outperforming of our approach.
Copyright © 2016 Nguyen Dang Binh, licensed to EAI. This is an open access article distributed under the terms of the Creative Commons Attribution licence (http://creativecommons.org/licenses/by/3.0/), which permits unlimited use, distribution and reproduction in any medium so long as the original work is properly cited.