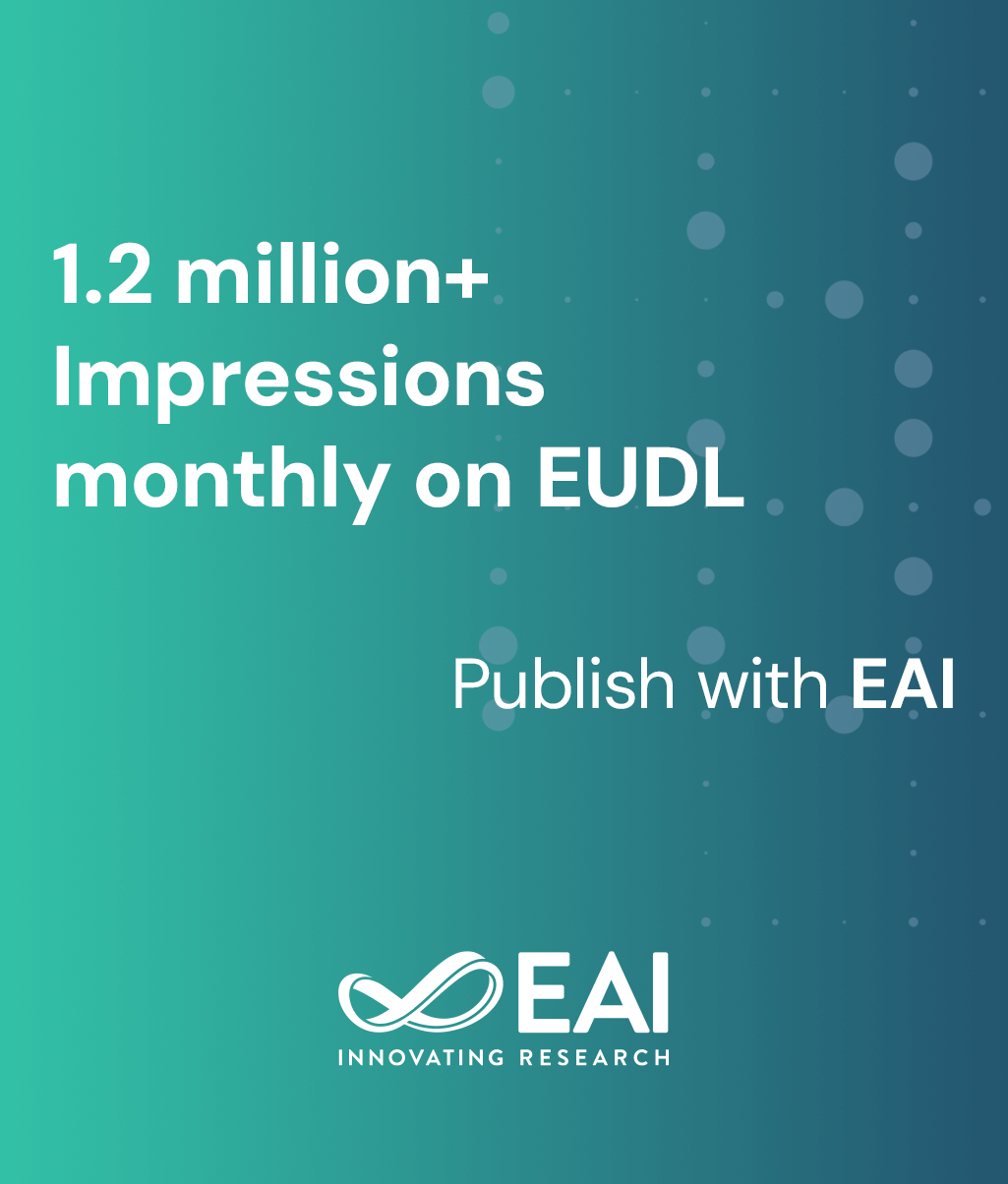
Research Article
Classification and analysis of spectrum sensing mechanisms in Cognitive Vehicular Networks
@ARTICLE{10.4108/eai.12-2-2018.154105, author={A. Riyahi and S. Bah and M. Sebgui and B. Elgraini}, title={Classification and analysis of spectrum sensing mechanisms in Cognitive Vehicular Networks}, journal={EAI Endorsed Transactions on Smart Cities}, volume={3}, number={7}, publisher={EAI}, journal_a={SC}, year={2018}, month={2}, keywords={Cognitive radio, CVNs, Spectrum sensing, Data fusion, Dense traffic, Correlation.}, doi={10.4108/eai.12-2-2018.154105} }
- A. Riyahi
S. Bah
M. Sebgui
B. Elgraini
Year: 2018
Classification and analysis of spectrum sensing mechanisms in Cognitive Vehicular Networks
SC
EAI
DOI: 10.4108/eai.12-2-2018.154105
Abstract
Vehicular Ad hoc Networks (VANETs) is an essential part of Intelligent Transportation System (ITS), which aims to improve the road safety. However, the main challenge in VANET is the spectrum scarcity which is more severe especially in the urban environment. In this view using Cognitive Radio (CR) technology in VANET has emerged as a promising solution providing additional resources and allowing spectrum efficiency. But, vehicular networks are highly challenging for spectrum sensing due to speed and dynamic topology. Furthermore, these parameters depend on the CVNs’ environment such as highway, urban or suburban. Therefore, solutions targeting CVNs should take into consideration these characteristics. As a first step towards an appropriate spectrum sensing solution for CVNs, we first, provide a comprehensive classification of existing spectrum sensing techniques for CVNs. Second, we discuss, for each class, the impact of the vehicular environment effects such as traffic density, speed and fading on the spectrum sensing and data fusion techniques. Thirdly, we derive a set of requirements for CVN’s spectrum sensing that takes into consideration specific characteristics of CVN environments. Finally, we propose a new CVN scheme adopted in particular for urban environment where the spectrum sensing is more challenging due to dense traffic and correlated shadowing.
Copyright © 2018 A. Riyahi et al., licensed to EAI. This is an open access article distributed under the terms of the Creative Commons Attribution licence (http://creativecommons.org/licenses/by/3.0/), which permits unlimited use, distribution and reproduction in any medium so long as the original work is properly cited.