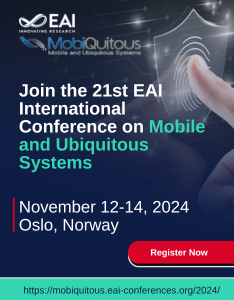
Research Article
Advanced High-order Hidden Bivariate Markov Model Based Spectrum Prediction
@ARTICLE{10.4108/eai.12-12-2017.153466, author={Yangxiao Zhao and Zhiming Hong and Yu Luo and Guodong Wang and Lina Pu}, title={Advanced High-order Hidden Bivariate Markov Model Based Spectrum Prediction}, journal={EAI Endorsed Transactions on Wireless Spectrum}, volume={3}, number={12}, publisher={EAI}, journal_a={WS}, year={2017}, month={12}, keywords={cognitive radio network, spectrum prediction, high-order hidden bivariate Markov model.}, doi={10.4108/eai.12-12-2017.153466} }
- Yangxiao Zhao
Zhiming Hong
Yu Luo
Guodong Wang
Lina Pu
Year: 2017
Advanced High-order Hidden Bivariate Markov Model Based Spectrum Prediction
WS
EAI
DOI: 10.4108/eai.12-12-2017.153466
Abstract
The majority of existing spectrum prediction models in Cognitive Radio Networks (CRNs) don’t fully explore the hidden correlation among adjacent observations. In this paper, we first develop a novel prediction approach termed high-order hidden bivariate Markov model (H2BMM) for a stationary CRN. The proposed H2BMM leverages the advantages of both HBMM and high-order, which applies two dimensional parameters, i.e., hidden process and underlying process, to more accurately describe the channel behavior. In addition, the current channel state is predicted by observing multiple previous states. Afterwards, the mobility of secondary users is fully considered and we propose an advanced approach based on H2BMM, termed Advanced H2BMM, to accommodate a mobile CRN. Extensive simulations are conducted and results verify that the prediction accuracy is significantly improved using the proposed (H2BMM. The Advanced H2BMM is also evaluated with comparison to H2BMM and results show considerable improvements of H2BMM in a mobile environment.
Copyright © 2017 Zhiming Hong et al., licensed to EAI. This is an open access article distributed under the terms of the Creative Commons Attribution license (http://creativecommons.org/licenses/by/3.0/), which permits unlimited use, distribution and reproduction in any medium so long as the original work is properly cited.