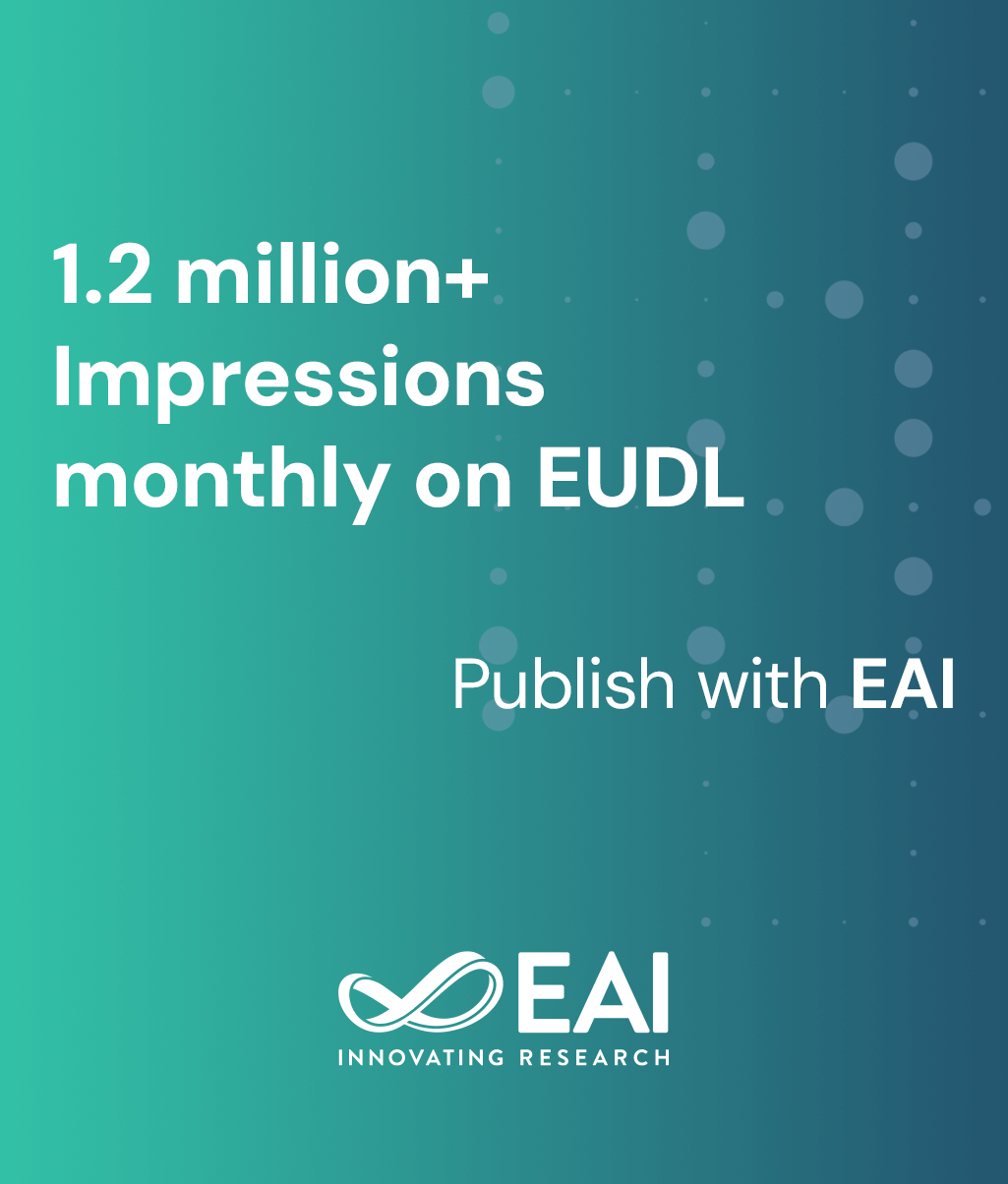
Research Article
Grouped Variable Model Selection for Heterogeneous Medical Signals
@INPROCEEDINGS{10.1145/2221924.2221962, author={Jamie Macbeth and Majid Sarrafzadeh}, title={Grouped Variable Model Selection for Heterogeneous Medical Signals}, proceedings={5th International ICST Conference on Body Area Networks}, publisher={ACM}, proceedings_a={BODYNETS}, year={2012}, month={6}, keywords={Medical Signals Regression Model Selection}, doi={10.1145/2221924.2221962} }
- Jamie Macbeth
Majid Sarrafzadeh
Year: 2012
Grouped Variable Model Selection for Heterogeneous Medical Signals
BODYNETS
ACM
DOI: 10.1145/2221924.2221962
Abstract
We explore statistical regression techniques for use in medical monitoring and telehealth applications. Medical embedded systems of the present and future are recording vast sets of data related to medical conditions and physiology. In this paper, distributed time-lag linear models are proposed as a means to help explain relationships between two or more medical and physiological measurements. The issues associated with performing multiple regression with heterogeneous medical data are treated as problems in model selection. An automatic method of model selection is proposed to construct models for high sample rate data by grouping sets of predictor variables.
The grouped predictor variable model optimization problem is formalized. Once an initial regression is performed on all available variables, our approximate algorithm for finding the grouped variable model with the greatest validity runs in $O(n^2)$ time, where $n$ is the number of available predictor variables. This is compared to the \textit{all subsets} technique which requires $O(2^n)$ time for the same predictor set. In our experiments with medical signal data, we find that the method produces models with reasonable goodness of fit scores and high average confidence levels for grouped predictors.