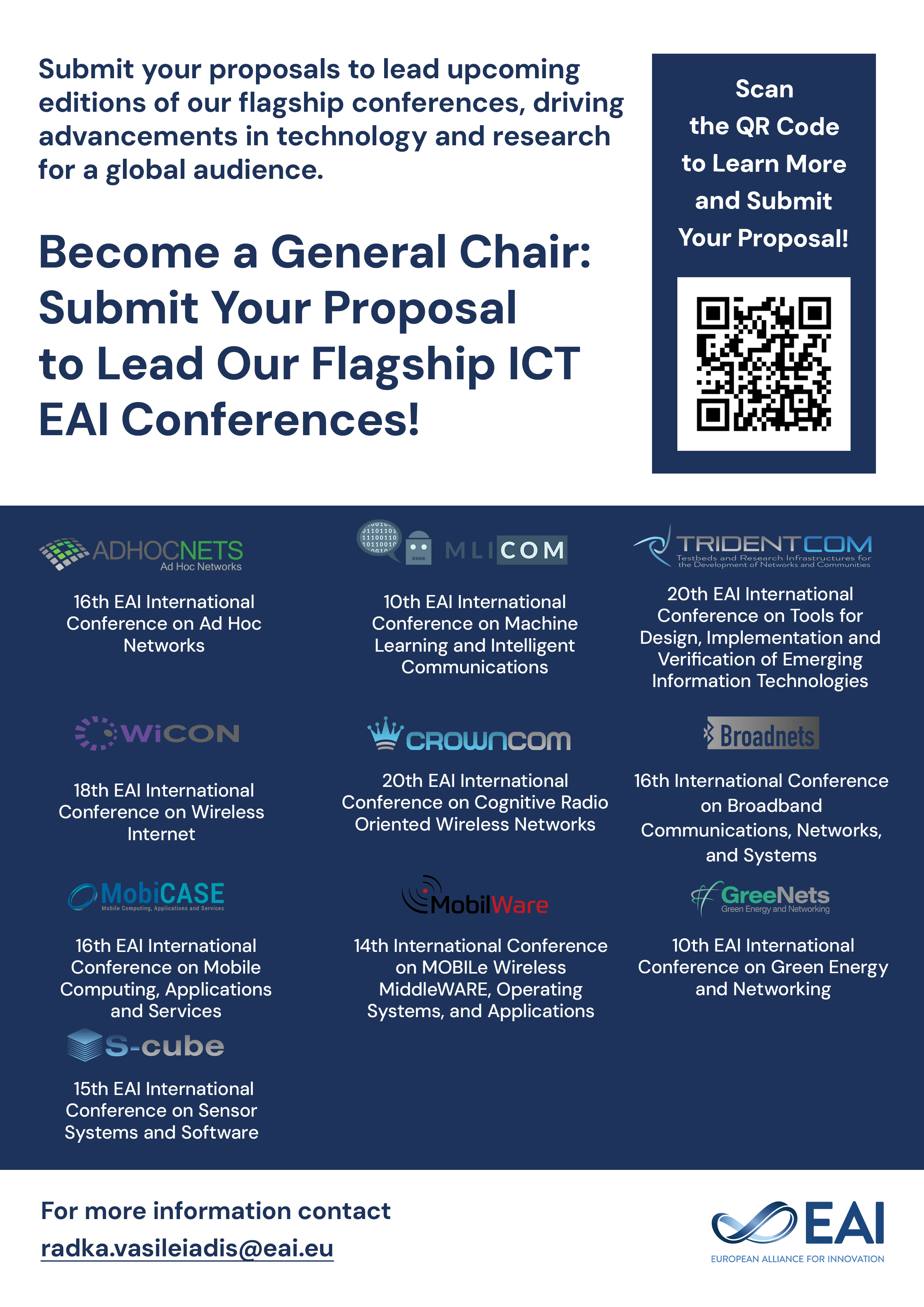
Research Article
Online learning in Markov decision processes with arbitrarily changing rewards and transitions
@INPROCEEDINGS{10.1109/GAMENETS.2009.5137416, author={Jia Yuan Yu and Shie Mannor}, title={Online learning in Markov decision processes with arbitrarily changing rewards and transitions}, proceedings={1st International Conference on Game Theory for Networks}, publisher={IEEE}, proceedings_a={GAMENETS}, year={2009}, month={6}, keywords={}, doi={10.1109/GAMENETS.2009.5137416} }
- Jia Yuan Yu
Shie Mannor
Year: 2009
Online learning in Markov decision processes with arbitrarily changing rewards and transitions
GAMENETS
IEEE
DOI: 10.1109/GAMENETS.2009.5137416
Abstract
We consider decision-making problems in Markov decision processes where both the rewards and the transition probabilities vary in an arbitrary (e.g., non-stationary) fashion. We present algorithms that combine online learning and robust control, and establish guarantees on their performance evaluated in retrospect against alternative policies-i.e., their regret. These guarantees depend critically on the range of uncertainty in the transition probabilities, but hold regardless of the changes in rewards and transition probabilities over time. We present a version of the main algorithm in the setting where the decision-maker's observations are limited to its trajectory, and another version that allows a trade-off between performance and computational complexity.