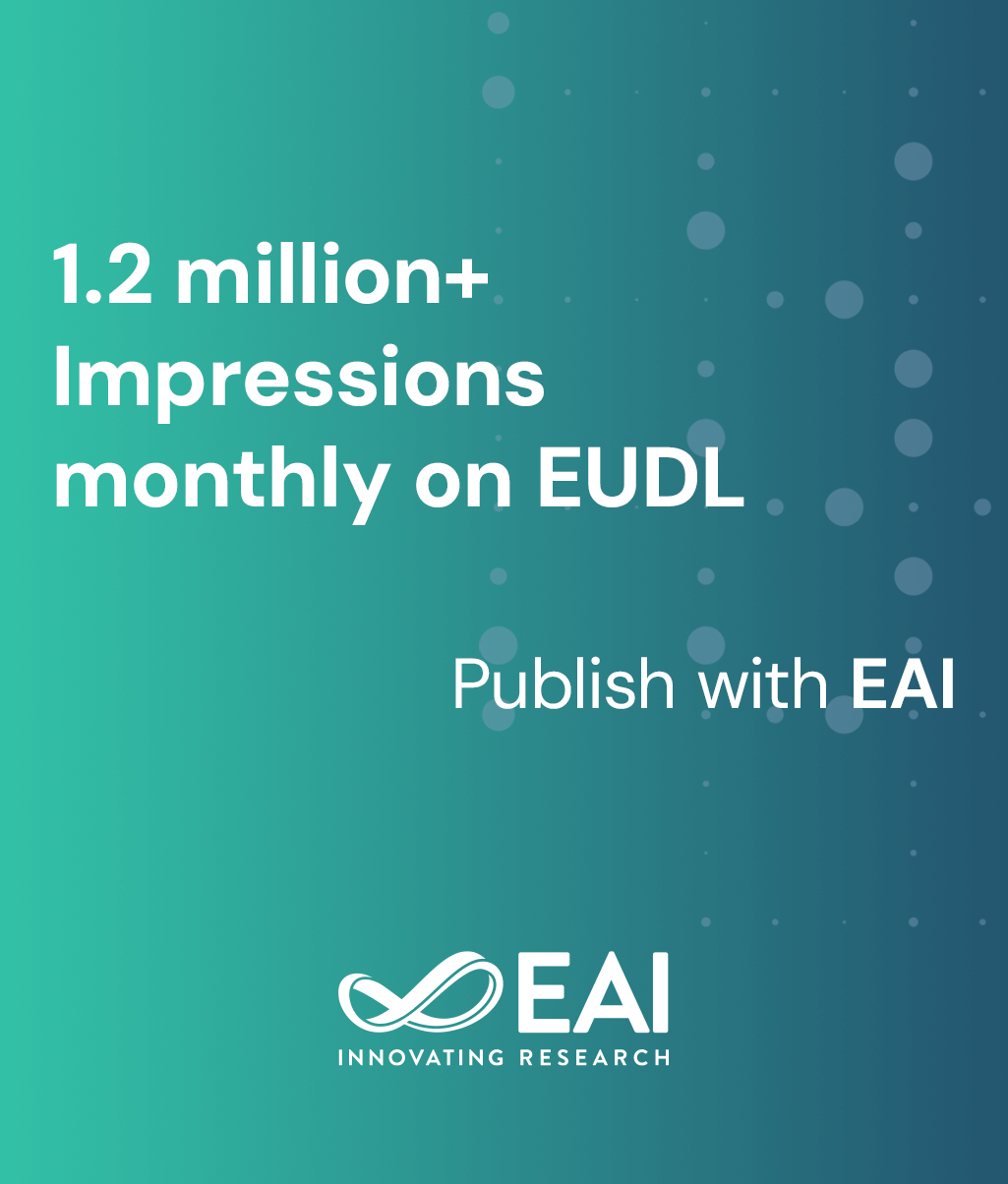
Research Article
Behavior Analysis of Spam Botnets
@INPROCEEDINGS{10.1109/COMSWA.2008.4554418, author={Husain Husna and Santi Phithakkitnukoon and Srikanth Palla and Ram Dantu}, title={Behavior Analysis of Spam Botnets}, proceedings={3rd International ICST Conference on COMmunication System SoftWAre and MiddlewaRE}, publisher={IEEE}, proceedings_a={COMSWARE}, year={2008}, month={6}, keywords={}, doi={10.1109/COMSWA.2008.4554418} }
- Husain Husna
Santi Phithakkitnukoon
Srikanth Palla
Ram Dantu
Year: 2008
Behavior Analysis of Spam Botnets
COMSWARE
IEEE
DOI: 10.1109/COMSWA.2008.4554418
Abstract
Compromised computers, known as bots, are the major source of spamming and their detection helps greatly improve control of unwanted traffic. In this work we investigate the behavior patterns of spammers based on their underlying similarities in spamming. To our knowledge, no work has been reported on identifying spam botnets based on spammers’ temporal characteristics. Our study shows that the relationship among spammers demonstrates highly clustering structures based on features such as Content length, Time of arrival, Frequency of email, Active Time, Inter-arrival Time, and Content Type. Although the dimensions of the collected feature set is low, we perform Principal Component Analysis (PCA) on feature set to identify the features which account for the maximum variance in the spamming patterns. Further, we calculate the proximity between different spammers and classify them into various groups. Each group represents similar proximity. Spammers in the same group inherit similar patterns of spamming a domain. For classification into Botnet groups, we use clustering algorithms such as Hierarchical and K-means.We identify Botnet spammers into a particular group with a precision of 90%.