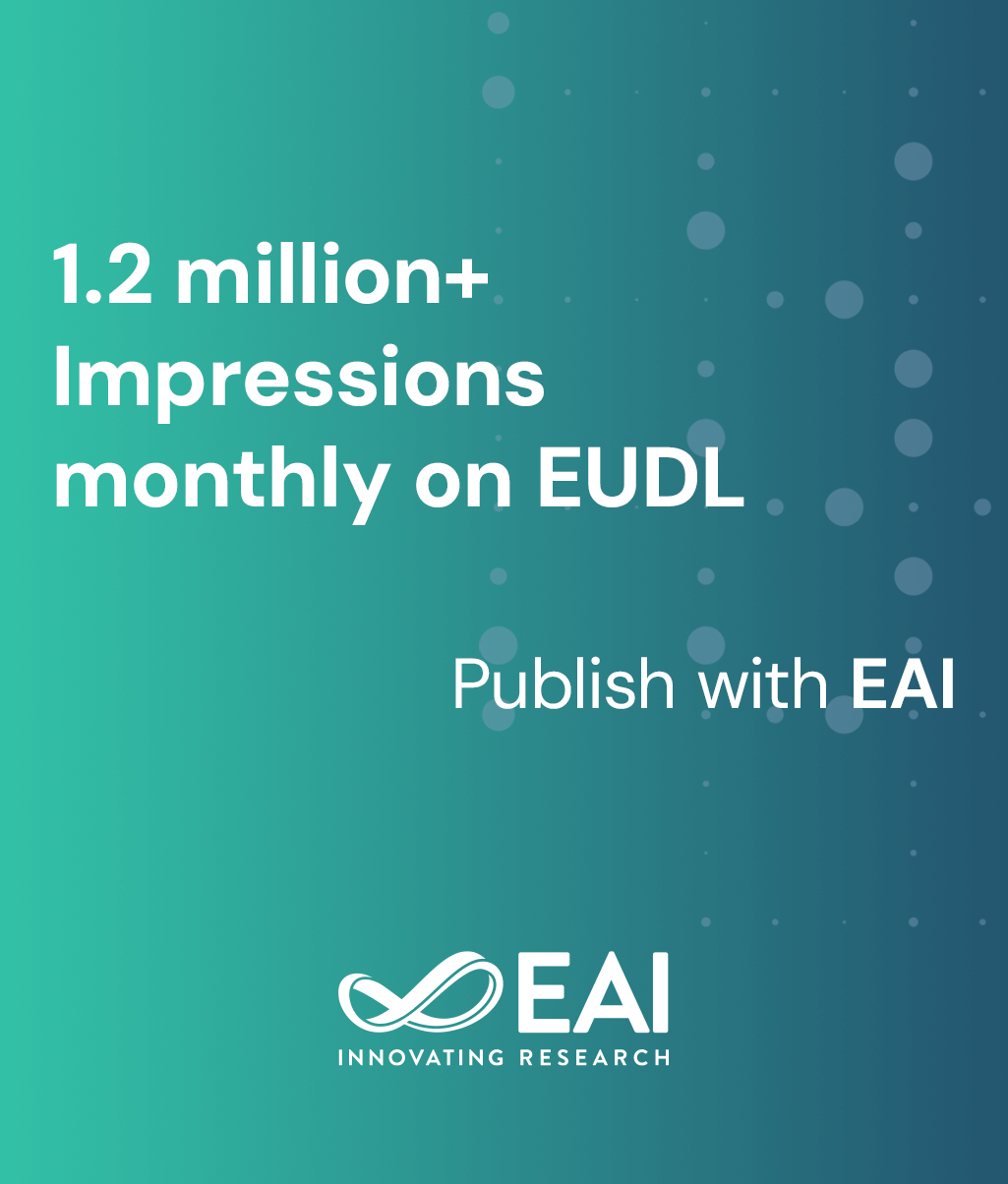
Research Article
Classification of Speech Dysfluencies Using Speech Parameterization Techniques and Multiclass SVM
@INPROCEEDINGS{10.1007/978-3-642-37949-9_26, author={P. Mahesha and D. Vinod}, title={Classification of Speech Dysfluencies Using Speech Parameterization Techniques and Multiclass SVM}, proceedings={Quality, Reliability, Security and Robustness in Heterogeneous Networks. 9th International Conference, QShine 2013, Greader Noida, India, January 11-12, 2013, Revised Selected Papers}, proceedings_a={QSHINE}, year={2013}, month={7}, keywords={}, doi={10.1007/978-3-642-37949-9_26} }
- P. Mahesha
D. Vinod
Year: 2013
Classification of Speech Dysfluencies Using Speech Parameterization Techniques and Multiclass SVM
QSHINE
Springer
DOI: 10.1007/978-3-642-37949-9_26
Abstract
Stuttering is a fluency disorder characterized by the occurrences of dysfluencies in normal flow of speech, such as repetitions, prolongations and interjection and so on. It is one of the serious problems in speech pathology. The goal of this paper is to present experimental results for the classification of three types of dysfluencies such as syllable repetition, word repetition and prolongation in stuttered speech. The three speech parameterization techniques :Linear Prediction Coefficients (LPC), Linear Prediction Cepstral Coefficients (LPCC) and Mel Frequency Cepstral Coefficients (MFCC) are used as speech feature extraction methods. The performance of these parameterization techniques are compared using the results obtained by thorough experimentation. The speech samples are obtained from University College London Archive of Stuttered Speech (UCLASS). The dysfluencies are extracted from these speech samples and used for feature extraction. The multi-class Support Vector Machine (SVM) is employed for the classification of speech dysfluencies.