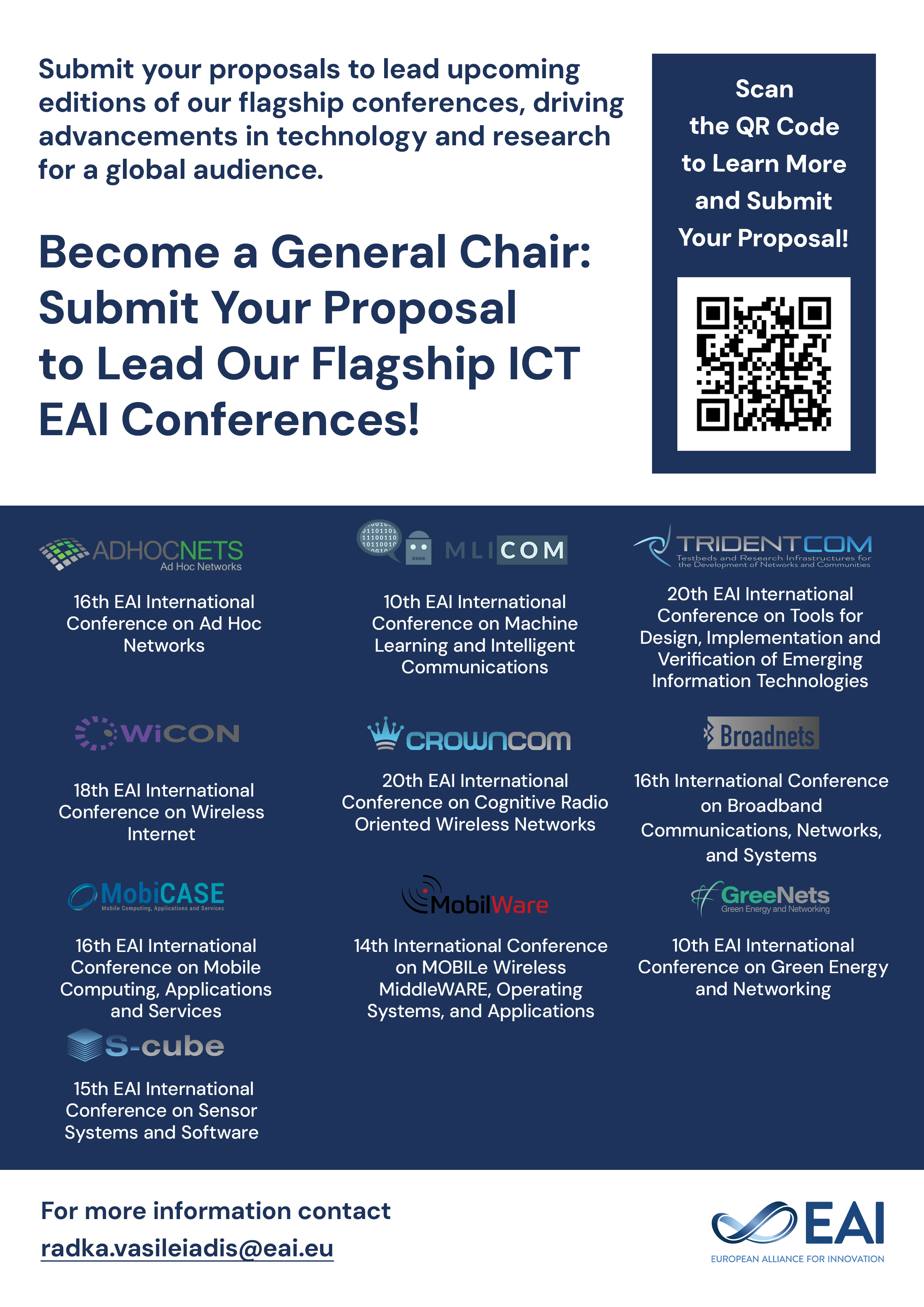
Research Article
A Competitive Rate Allocation Game
@INPROCEEDINGS{10.1007/978-3-642-35582-0_2, author={Yanting Wu and George Rabanca and Bhaskar Krishnamachari and Amotz Bar-Noy}, title={A Competitive Rate Allocation Game}, proceedings={Game Theory for Networks. Third International ICST Conference, GameNets 2012, Vancouver, BC, Canada, May 24-26, 2012, Revised Selected Papers}, proceedings_a={GAMENETS}, year={2012}, month={12}, keywords={competitive rate allocation game Nash equilibrium online learning}, doi={10.1007/978-3-642-35582-0_2} }
- Yanting Wu
George Rabanca
Bhaskar Krishnamachari
Amotz Bar-Noy
Year: 2012
A Competitive Rate Allocation Game
GAMENETS
Springer
DOI: 10.1007/978-3-642-35582-0_2
Abstract
We introduce a competitive rate allocation game in which two receivers compete to forward the data from a transmitter to a destination in exchange for a payment proportional to the amount of forwarded data. At each time slot the channel from the transmitter to each receiver is an independent random variable with two states, high or low, affecting the amount of data that can be transmitted. Receivers make ”bids” on the state of their channel and the transmitter allocates rate accordingly. Receivers are rewarded for successful transmissions and penalized for unsuccessful transmissions. The goal of the transmitter is to set the penalties in such a way that even if the receivers are selfish, the data forwarded is close to the optimal transmission rate. We first model this problem as a single shot game in which the receivers know the channel probability distributions but the transmitter does not, and show that it is possible for the transmitter to set penalties so as to ensure that both receivers have a dominant strategy and the corresponding Price of Anarchy is bounded by 2. We show, moreover, that this is in a sense the best possible bound. We next consider the case when receivers have incomplete information on the distributions, and numerically evaluate the performance of a distributed online learning algorithm based on the well-known UCB1 policy for this case. From simulations, we find that use of UCB1 policy yields a performance close to the dominant strategy.