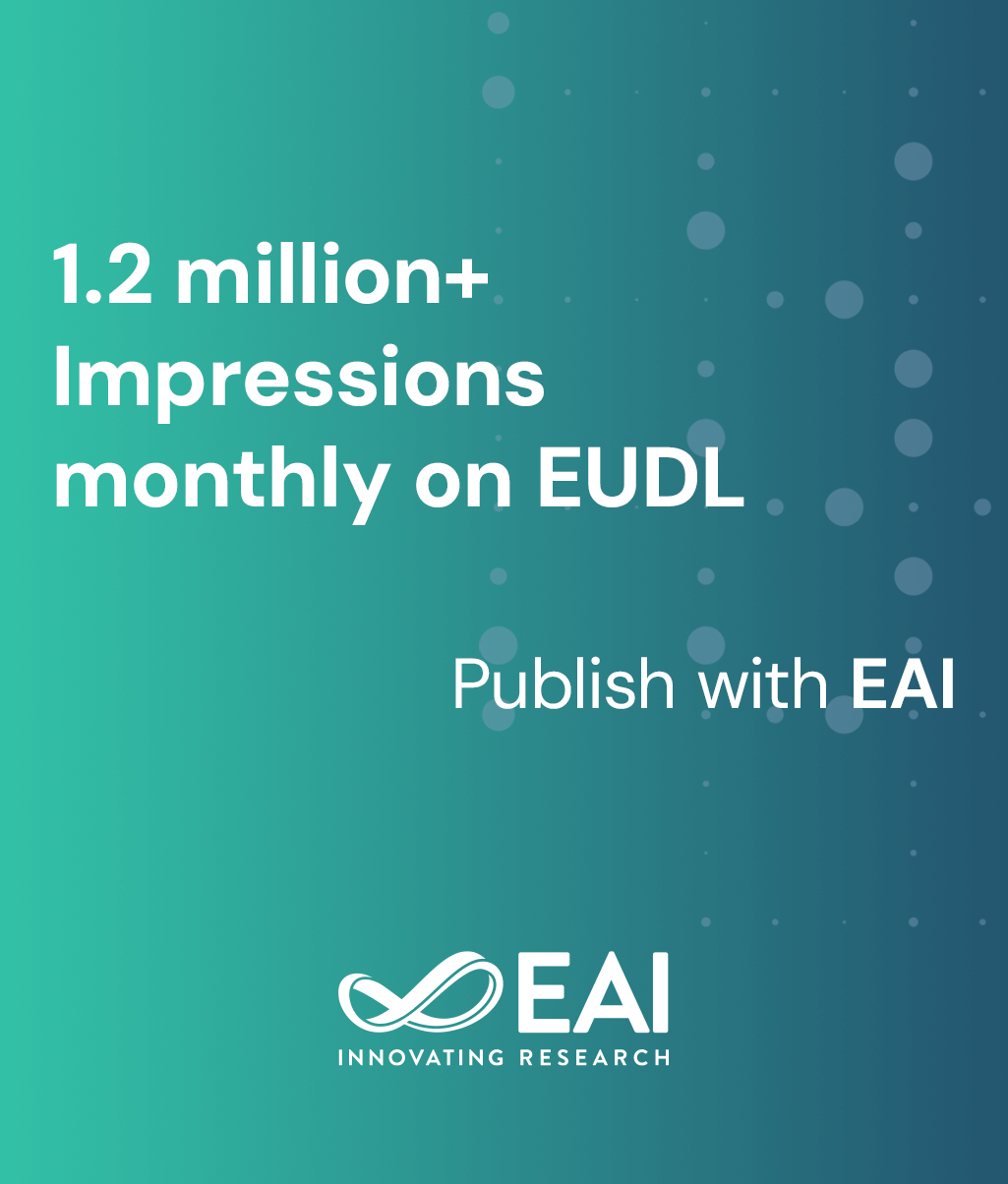
Research Article
Algorithmically Transitive Network: A Self-organizing Data-Flow Network with Learning
@INPROCEEDINGS{10.1007/978-3-642-32615-8_9, author={Hideaki Suzuki and Hiroyuki Ohsaki and Hidefumi Sawai}, title={Algorithmically Transitive Network: A Self-organizing Data-Flow Network with Learning}, proceedings={Bio-Inspired Models of Network, Information, and Computing Systems. 5th International ICST Conference, BIONETICS 2010, Boston, USA, December 1-3, 2010, Revised Selected Papers}, proceedings_a={BIONETICS}, year={2012}, month={10}, keywords={data-flow network learning neural network back-propagation artificial chemistry}, doi={10.1007/978-3-642-32615-8_9} }
- Hideaki Suzuki
Hiroyuki Ohsaki
Hidefumi Sawai
Year: 2012
Algorithmically Transitive Network: A Self-organizing Data-Flow Network with Learning
BIONETICS
Springer
DOI: 10.1007/978-3-642-32615-8_9
Abstract
A novel non-von Neumann computational model named “Algorithmically Transitive Network” (ATN) is presented. The ATN is a data-flow network composed of operation nodes and data edges. The calculation is propelled with node firing and token creation on the edges. After it finishes, teaching values are given to the answer nodes and an energy function is evaluated, which causes backward propagation of differential coefficients with respect to token variables or node parameters. The network’s topological alteration takes place based on these calculation/learning processes, and as a result, the algorithm of the network is refined. The basic scheme of the model is explained, and some experimental results on symbolic regression problems are presented.