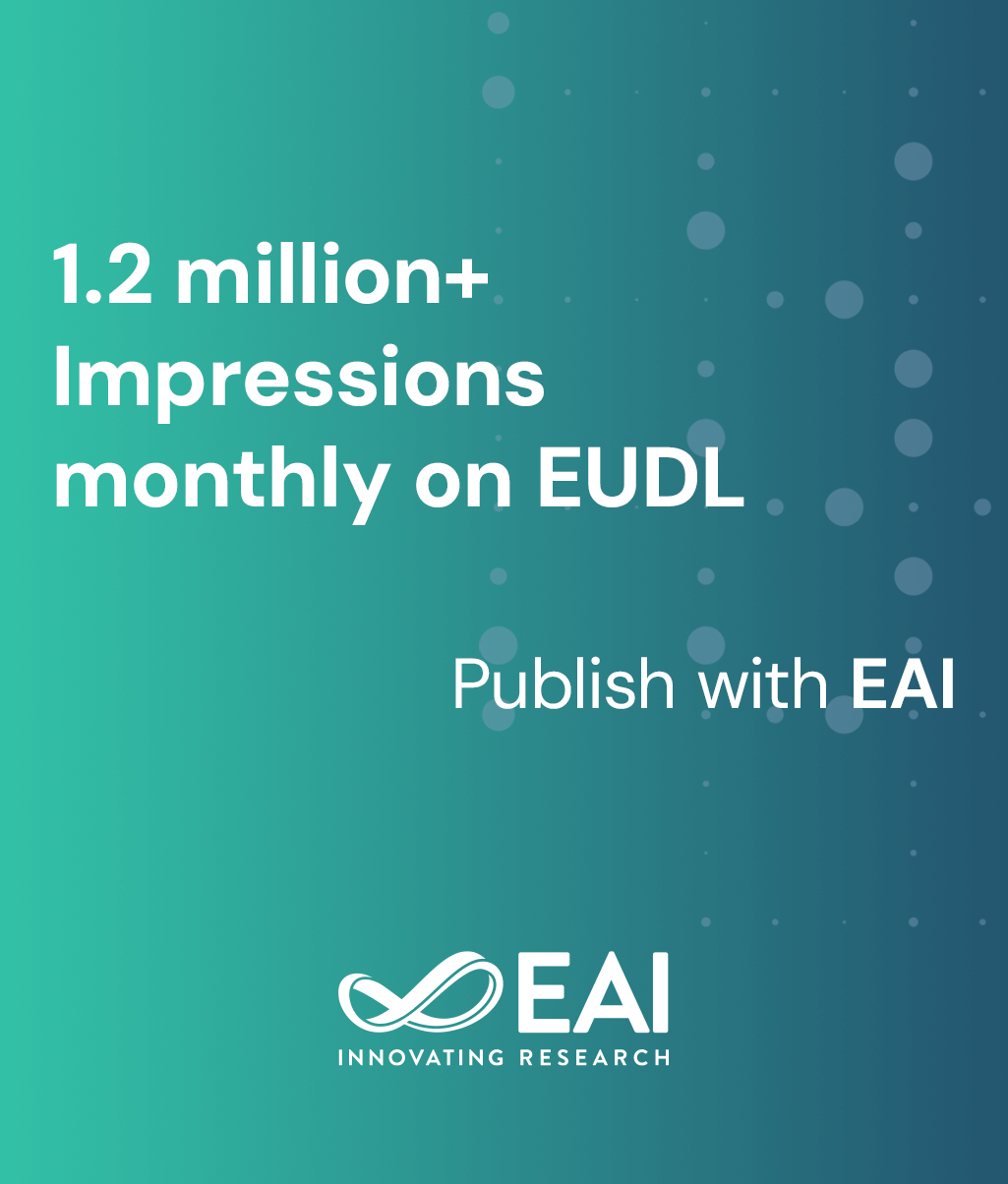
Research Article
Sensor Based Time Series Classification of Body Movement
@INPROCEEDINGS{10.1007/978-3-642-32615-8_30, author={Swapna Philip and Yu Cao and Ming Li}, title={Sensor Based Time Series Classification of Body Movement}, proceedings={1st Workshop on Bio-inspired Models and Technologies for Ambient Information Society}, proceedings_a={BIOAMBIS}, year={2012}, month={10}, keywords={Body Sensor Networks Time Series Analysis Human body movement machine learning classification}, doi={10.1007/978-3-642-32615-8_30} }
- Swapna Philip
Yu Cao
Ming Li
Year: 2012
Sensor Based Time Series Classification of Body Movement
BIOAMBIS
Springer
DOI: 10.1007/978-3-642-32615-8_30
Abstract
Advances in sensing and monitoring technology are being incorporated into today’s healthcare practice. As a result, the concept of Body Sensor Networks (BSN) has been proposed to describe the wearable/wireless devices for healthcare applications. One of the major application scenarios for BSN is to detect and classify the body movements for long-term lifestyle and healthcare monitoring. This paper introduces a new approach for analyzing the time series obtained from BSN. In our research, the BSN record the acceleration data of the volunteer’s movement while performing a set of activities such as jogging, walking, resting, and transitional activities. The main contribution of this paper is the proposed time series approximation and feature extraction algorithm that can convert the sensor-based time series data into a density map. We have performed extensive experiments to compare the accuracy in classifying the time series into different activities. It is concluded that the proposed approach would aid greatly the development of efficient health monitoring systems in the future. To the best of our knowledge, no similar research has been reported in the BSN field and we expect our research could provide useful insights for further investigation.