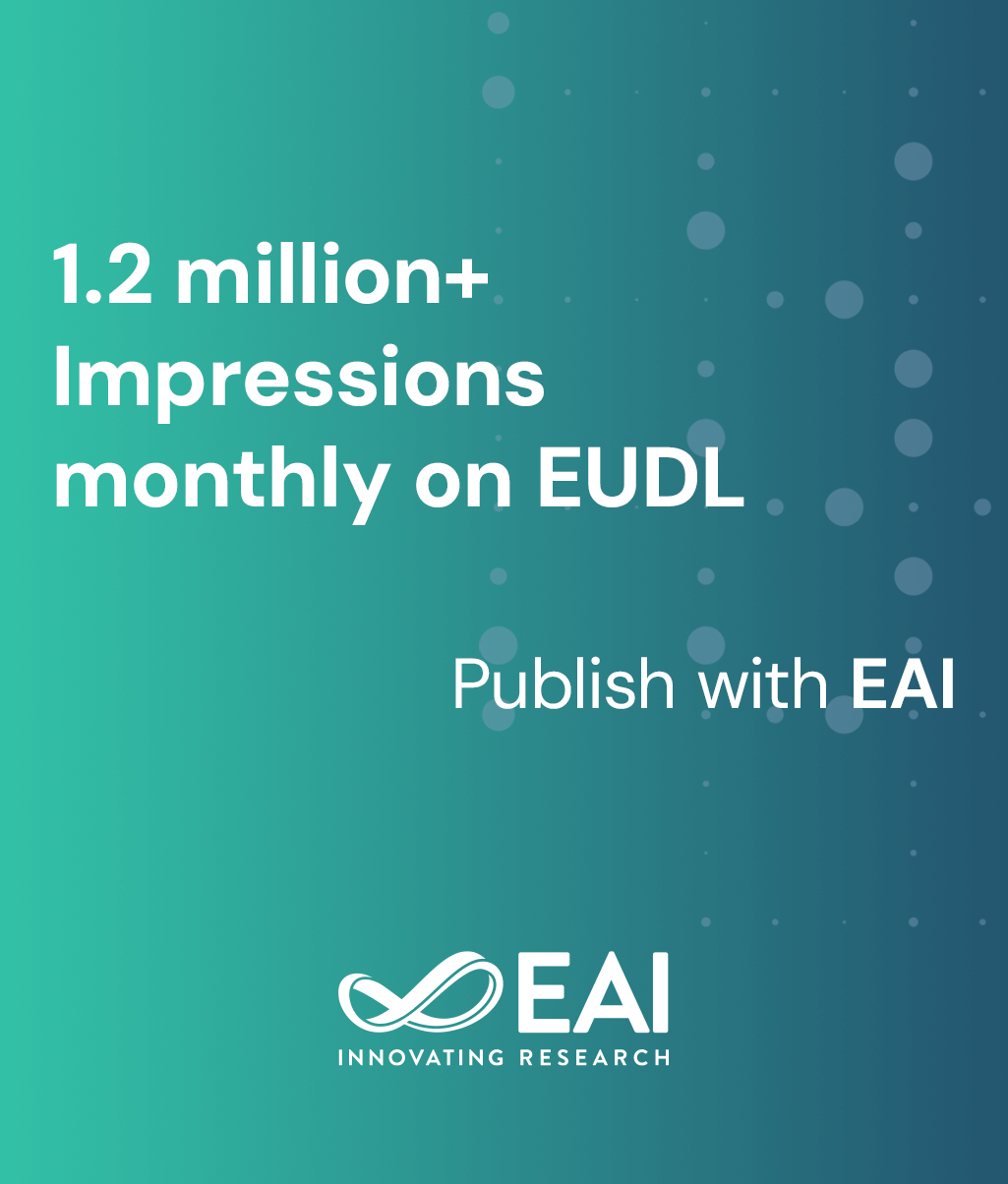
Research Article
A Sparse Bayesian Framework for Anomaly Detection in Heterogeneous Networks
@INPROCEEDINGS{10.1007/978-3-642-29222-4_6, author={Jian Zhang and Rajgopal Kannan}, title={A Sparse Bayesian Framework for Anomaly Detection in Heterogeneous Networks}, proceedings={Quality, Reliability, Security and Robustness in Heterogeneous Networks. 7th International Conference on Heterogeneous Networking for Quality, Reliability, Security and Robustness, QShine 2010, and Dedicated Short Range Communications Workshop, DSRC 2010, Houston, TX, USA, November 17-19, 2010, Revised Selected Papers}, proceedings_a={QSHINE}, year={2012}, month={10}, keywords={Anomaly Detection Sparse Bayesian Classification}, doi={10.1007/978-3-642-29222-4_6} }
- Jian Zhang
Rajgopal Kannan
Year: 2012
A Sparse Bayesian Framework for Anomaly Detection in Heterogeneous Networks
QSHINE
Springer
DOI: 10.1007/978-3-642-29222-4_6
Abstract
The capability to detect anomalous states in a network is important for both the smooth operation of the network and the security of the network. Modern networks are often heterogeneous. This raises a new challenge for anomaly detection, as there may be a wide variety of anomalous activities across the heterogeneous components of a network. We often seek a detection system that not only performs accurate anomaly detection but also provides mechanisms for human expert to understand the decision making process inside the system. In this paper, we investigate the application of sparse Bayesian methods for anomaly detection in such scenario. By taking advantage of the sparse Bayesian framework’s capability to conduct automatic relevance discovery, we construct a detection system whose decision making is mostly based on a few representative examples from the training set. This provides human interpretability as expert can analyze the representative examples to understand the detection mechanism. Our experiment results show the potential of this approach.