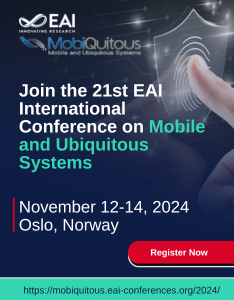
Research Article
Towards a Framework for Privacy Preserving Medical Data Mining Based on Standard Medical Classifications
@INPROCEEDINGS{10.1007/978-3-642-23635-8_26, author={Aur\^{e}lien Faravelon and Christine Verdier}, title={Towards a Framework for Privacy Preserving Medical Data Mining Based on Standard Medical Classifications}, proceedings={Electronic Healthcare. Third International Conference, eHealth 2010, Casablanca, Morocco, December 13-15, 2010, Revised Selected Papers}, proceedings_a={E-HEALTH}, year={2012}, month={10}, keywords={Privacy Data Pattern Hidding Data Generalisation}, doi={10.1007/978-3-642-23635-8_26} }
- Aurélien Faravelon
Christine Verdier
Year: 2012
Towards a Framework for Privacy Preserving Medical Data Mining Based on Standard Medical Classifications
E-HEALTH
Springer
DOI: 10.1007/978-3-642-23635-8_26
Abstract
Privacy-preserving data mining often focuses on data alteration but may bias data patterns interpretation and does not offer different levels of access to patterns according to their use. This paper addresses data mining as a prediction tool and proposes to offer several levels of access to data patterns according to users’ trustworthiness. The grounding intuition is that patterns’ predictive value depends on their precision that should thus vary according to their use. The following problem is considered: a medical data holder wants to disclose data or data patterns and still control the meaning of the disclosed patterns or of the patterns that may be mined out of the released dataset. To tackle this issue, we propose a framework compliant with existing data mining techniques by modeling trust in terms of data precision and generalising data according to standard medical classifications.