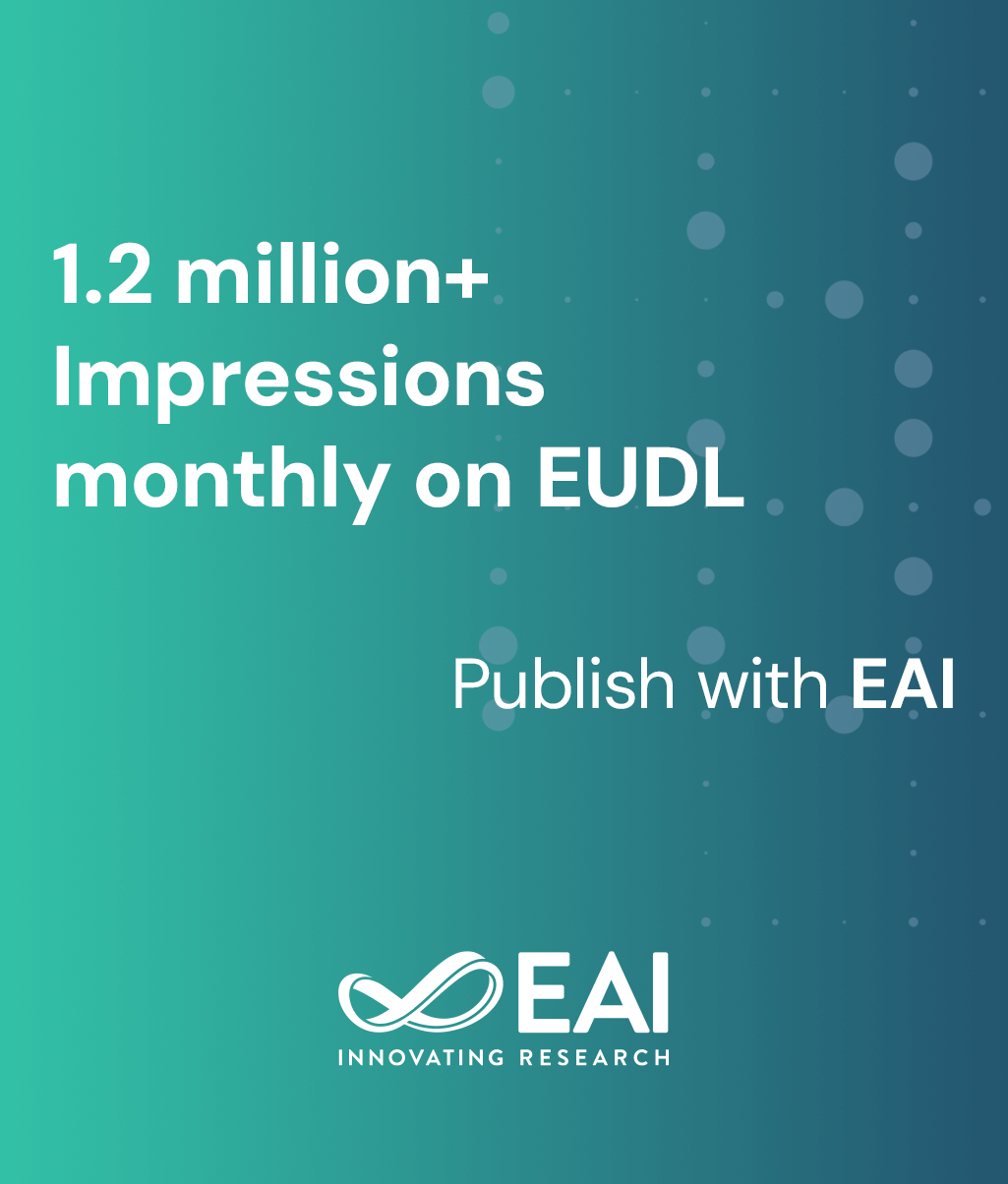
Research Article
On Assessing Motor Disorders in Parkinson’s Disease
@INPROCEEDINGS{10.1007/978-3-642-20865-2_5, author={Markos Tsipouras and Alexandros Tzallas and Evanthia Tripoliti and Georgios Rigas and Panagiota Bougia and Dimitrios Fotiadis and Sofia Tsouli and Spyridon Konitsiotis}, title={On Assessing Motor Disorders in Parkinson’s Disease}, proceedings={Wireless Mobile Communication and Healthcare. Second International ICST Conference, MobiHealth 2010, Ayia Napa, Cyprus, October 18-20, 2010. Revised Selected Papers}, proceedings_a={MOBIHEALTH}, year={2012}, month={5}, keywords={Parkinson’s disease motor symptoms assessment Levodopa-induced dyskinesia Freezing of Gait accelerometer gyroscope}, doi={10.1007/978-3-642-20865-2_5} }
- Markos Tsipouras
Alexandros Tzallas
Evanthia Tripoliti
Georgios Rigas
Panagiota Bougia
Dimitrios Fotiadis
Sofia Tsouli
Spyridon Konitsiotis
Year: 2012
On Assessing Motor Disorders in Parkinson’s Disease
MOBIHEALTH
Springer
DOI: 10.1007/978-3-642-20865-2_5
Abstract
In this paper we propose an automated method for assessing motor symptoms in Parkinson’s disease. Levodopa-induced dyskinesia (LID) and Freezing of Gait (FoG) are detected based on the analysis of signals recorded from wearable devices, i.e. accelerometers and gyroscopes, which are placed on certain positions on the patient’s body. The signals are initially pre-processed and then analyzed, using a moving window, in order to extract features from them. These features are used for LID and FoG assessment. Two classification techniques are employed, decision trees and random forests. The method has been evaluated using a group of patients and the obtained results indicate high classification ability, being 96.11% classification accuracy for FoG detection and 92.59% for LID severity assessment.