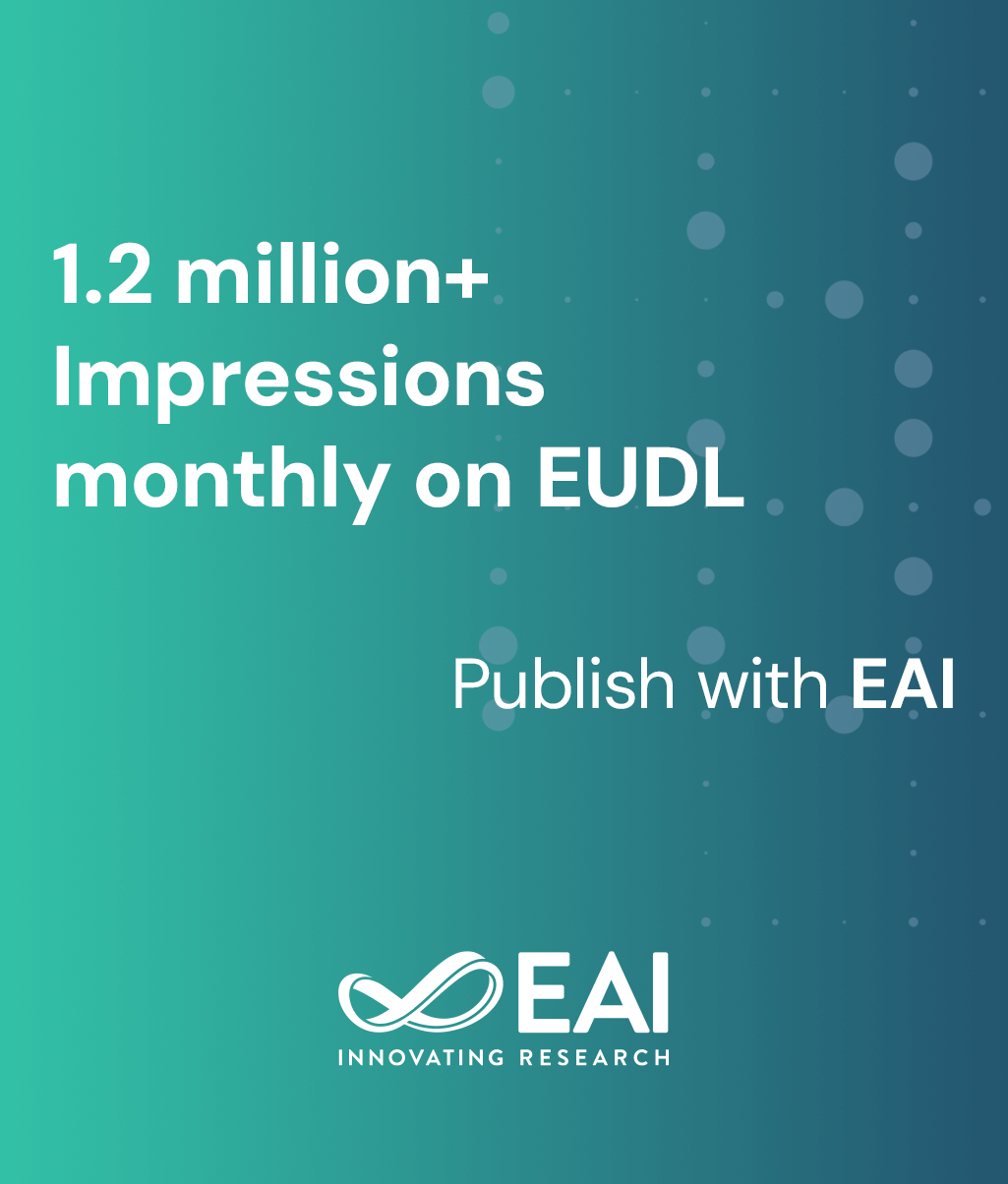
Research Article
Predictive Metabolic Modeling for Type 1 Diabetes Using Free-Living Data on Mobile Devices
@INPROCEEDINGS{10.1007/978-3-642-20865-2_24, author={Eleni Georga and Vasilios Protopappas and Dimitrios Fotiadis}, title={Predictive Metabolic Modeling for Type 1 Diabetes Using Free-Living Data on Mobile Devices}, proceedings={Wireless Mobile Communication and Healthcare. Second International ICST Conference, MobiHealth 2010, Ayia Napa, Cyprus, October 18-20, 2010. Revised Selected Papers}, proceedings_a={MOBIHEALTH}, year={2012}, month={5}, keywords={glucose prediction type 1 diabetes compartmental models support vector regression}, doi={10.1007/978-3-642-20865-2_24} }
- Eleni Georga
Vasilios Protopappas
Dimitrios Fotiadis
Year: 2012
Predictive Metabolic Modeling for Type 1 Diabetes Using Free-Living Data on Mobile Devices
MOBIHEALTH
Springer
DOI: 10.1007/978-3-642-20865-2_24
Abstract
This study presents a metabolic modeling scheme for glucose prediction of diabetic patients that is intended for use in mobile devices. We investigate the ability to model the multivariate, nonlinear and dynamic interactions in glucose metabolism using free-living data acquired from wearable sensors or inserted through suitable mobile applications. The physiological processes related to diabetes are simulated by compartmental models, which quantify the absorption of subcutaneously administered insulin, the absorption of glucose from the gut following a meal, as well as the effects of exercise on plasma glucose and insulin dynamics. In addition, Support Vector machines for Regression are employed to provide individualized predictions of the subcutaneous glucose concentrations. The proposed scheme is evaluated in terms of its predictive ability using real data recorded from two type 1 diabetic patients. Also, the incorporation of the predictive model in an integrated diabetes monitoring and management system is discussed.