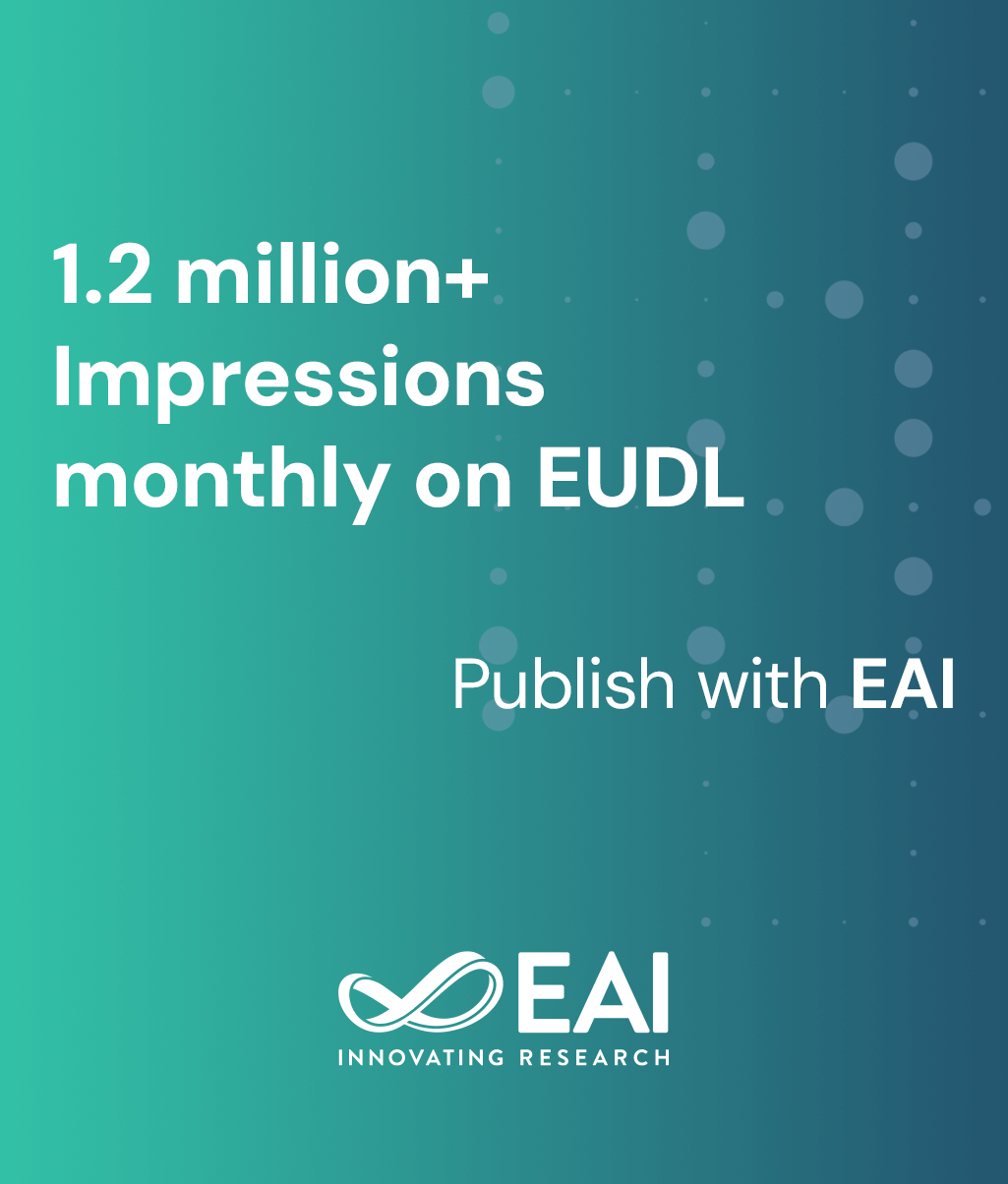
Research Article
Applying Behavioral Detection on Android-Based Devices
@INPROCEEDINGS{10.1007/978-3-642-17758-3_17, author={Asaf Shabtai and Yuval Elovici}, title={Applying Behavioral Detection on Android-Based Devices}, proceedings={Mobile Wireless Middleware, Operating Systems, and Applications. Third International Conference, Mobilware 2010, Chicago, IL, USA, June 30 - July 2, 2010. Revised Selected Papers}, proceedings_a={MOBILWARE}, year={2012}, month={10}, keywords={Intrusion Detection Mobile Devices Machine Learning Malware Security Android}, doi={10.1007/978-3-642-17758-3_17} }
- Asaf Shabtai
Yuval Elovici
Year: 2012
Applying Behavioral Detection on Android-Based Devices
MOBILWARE
Springer
DOI: 10.1007/978-3-642-17758-3_17
Abstract
We present Andromaly - a behavioral-based detection framework for Android-powered mobile devices. The proposed framework realizes a Host-based Intrusion Detection System (HIDS) that continuously monitors various features and events obtained from the mobile device, and then applies Machine Learning methods to classify the collected data as normal (benign) or abnormal (malicious). Since no malicious applications are yet available for Android, we evaluated Andromaly’s ability to differentiate between game and tool applications. Successful differentiation between games and tools is expected to provide a positive indication about the ability of such methods to learn and model the behavior of an Android application and potentially detect malicious applications. Several combinations of classification algorithms, feature selections and the number of top features were evaluated. Empirical results suggest that the proposed detection framework is effective in detecting types of applications having similar behavior, which is an indication for the ability to detect unknown malware in the Android framework.