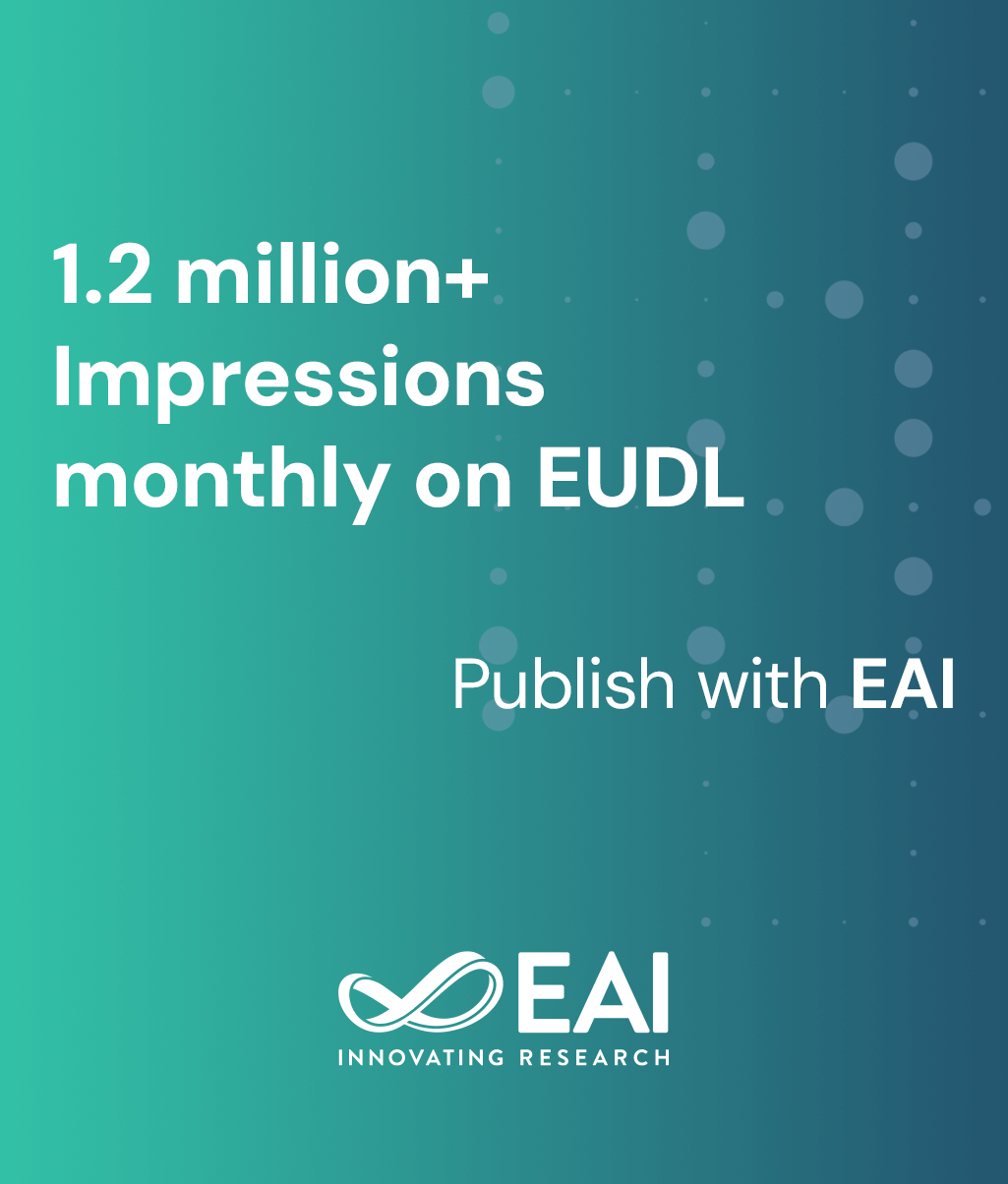
Research Article
Digital Evidence Composition in Fraud Detection
@INPROCEEDINGS{10.1007/978-3-642-11534-9_1, author={Sriram Raghavan and S. Raghavan}, title={Digital Evidence Composition in Fraud Detection}, proceedings={Digital Forensics and Cyber Crime. First International ICST Conference, ICDF2C 2009, Albany, NY, USA, September 30-October 2, 2009, Revised Selected Papers}, proceedings_a={ICDF2C}, year={2012}, month={5}, keywords={Evidence source Event Correlation function Probability function}, doi={10.1007/978-3-642-11534-9_1} }
- Sriram Raghavan
S. Raghavan
Year: 2012
Digital Evidence Composition in Fraud Detection
ICDF2C
Springer
DOI: 10.1007/978-3-642-11534-9_1
Abstract
In recent times, digital evidence has found its way into several digital devices. The storage capacity in these devices is also growing exponentially. When investigators come across such devices during a digital investigation, it may take several man-hours to completely analyze the contents. To date, there has been little achieved in the zone that attempts to bring together different evidence sources and attempt to correlate the events they record. In this paper, we present an evidence composition model based on the time of occurrence of such events. The time interval between events promises to reveal many key associations across events, especially when on multiple sources. The time interval is then used as a parameter to a correlation function which determines quantitatively the extent of correlation between the events. The approach has been demonstrated on a network capture sequence involving phishing of a bank website. The model is scalable to an arbitrary set of evidence sources and preliminary results indicate that the approach has tremendous potential in determining correlations on vast repositories of case data.