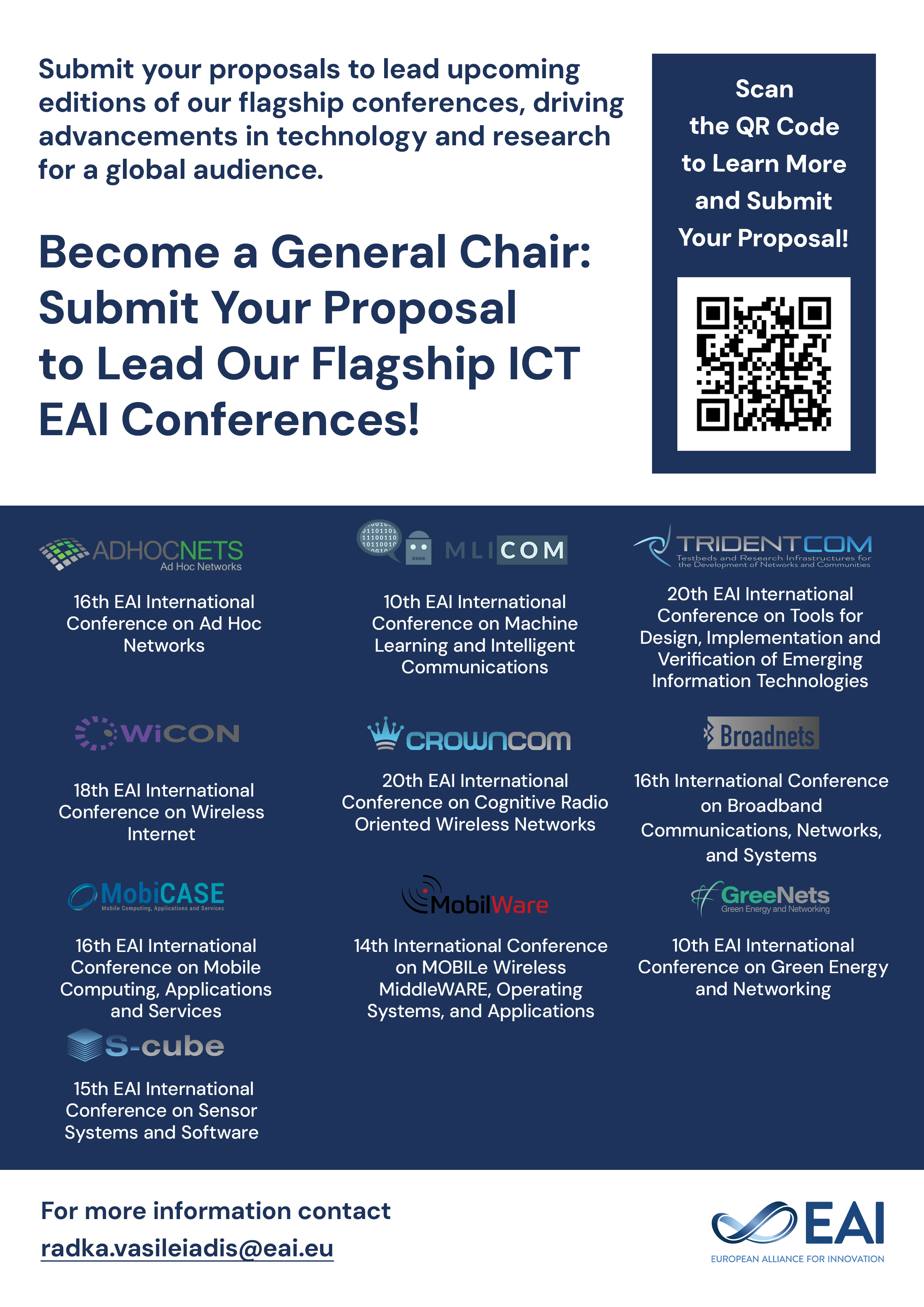
Research Article
Resource-Optimized Quality-Assured Ambiguous Context Mediation in Pervasive Environments
@INPROCEEDINGS{10.1007/978-3-642-10625-5_15, author={Nirmalya Roy and Christine Julien and Sajal Das}, title={Resource-Optimized Quality-Assured Ambiguous Context Mediation in Pervasive Environments}, proceedings={Quality of Service in Heterogeneous Networks. 6th International ICST Conference on Heterogeneous Networking for Quality, Reliability, Security and Robustness, QShine 2009 and 3rd International Workshop on Advanced Architectures and Algorithms for Internet Delivery and Applications, AAA-IDEA 2009, Las Palmas, Gran Canaria, November 23-25, 2009 Proceedings}, proceedings_a={QSHINE}, year={2012}, month={10}, keywords={Context-awareness Ambiguous contexts Bayesian networks Multi sensor fusion Information theory SunSPOT}, doi={10.1007/978-3-642-10625-5_15} }
- Nirmalya Roy
Christine Julien
Sajal Das
Year: 2012
Resource-Optimized Quality-Assured Ambiguous Context Mediation in Pervasive Environments
QSHINE
Springer
DOI: 10.1007/978-3-642-10625-5_15
Abstract
Pervasive computing applications envision sensor rich computing and networking environments that can capture various types of contexts of inhabitants of the environment, such as their locations, activities, vital signs, and environmental measures. Such context information is useful in a variety of applications, for example to manage health information to promote independent living in “aging-in-place” scenarios. In reality, both sensed and interpreted contexts are often ambiguous, leading to potentially dangerous decisions if not properly handled. Thus, a significant challenge facing the development of realistic and deployable context-aware services for pervasive computing applications is the ability to deal with these ambiguous contexts. In this paper, we propose a resource optimized quality assured context mediation framework for resource constrained sensor networks based on efficient context-aware data fusion and information theoretic sensor parameter selection for optimal state estimation. The proposed framework provides a systematic approach based on dynamic Bayesian networks to derive context fragments and deal with context ambiguity or error in a probabilistic manner. Experimental results using SunSPOT sensors demonstrate the promise of this approach.