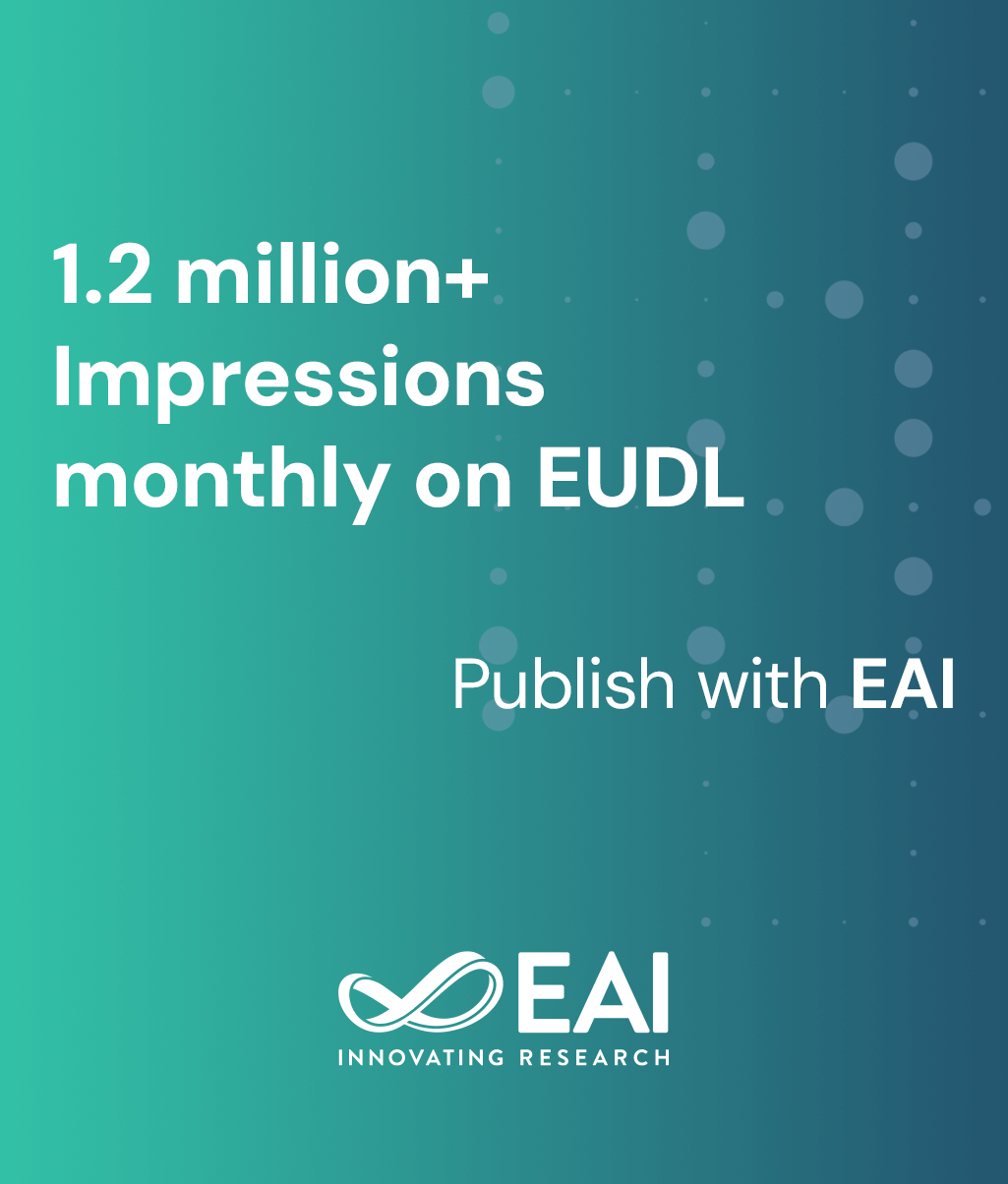
Research Article
Examining a Bayesian Approach to Personalizing Context Awareness in Ubiquitous Computing Environments
@INPROCEEDINGS{10.1007/978-3-642-03354-4_5, author={Yaser Mowafi and Dongsong Zhang and Guisseppi Fogionne}, title={Examining a Bayesian Approach to Personalizing Context Awareness in Ubiquitous Computing Environments}, proceedings={Collaborative Computing: Networking, Applications and Worksharing. 4th International Conference, CollaborateCom 2008, Orlando, FL, USA, November 13-16, 2008, Revised Selected Papers}, proceedings_a={COLLABORATECOM}, year={2012}, month={5}, keywords={Context-aware computing mobile and ubiquitous computing context modeling context inference context history machine learning Bayesian networks}, doi={10.1007/978-3-642-03354-4_5} }
- Yaser Mowafi
Dongsong Zhang
Guisseppi Fogionne
Year: 2012
Examining a Bayesian Approach to Personalizing Context Awareness in Ubiquitous Computing Environments
COLLABORATECOM
Springer
DOI: 10.1007/978-3-642-03354-4_5
Abstract
There is a growing interest in the use of context-aware systems, which can recognize user’s situational context and accordingly provide a desirable interaction with the user. However, the design, development, evaluation and deployment of context-aware systems are still at its infancy. In this paper, we propose an operational mechanism approach to context acquisition driven by the relevant dependency between users’ ongoing activities and context in ubiquitous computing environments. We present a Bayesian model to classify and predict users’ activities and associated context that offers more interactive and construed context awareness to users. The model performance is examined in terms of its classification accuracy in predicting users’ activities and the associated context trends. The results demonstrate that such probabilistic models can provide an effective and feasible approach to learning and predicting users’ context that can yield further the development of deployable context-aware systems.