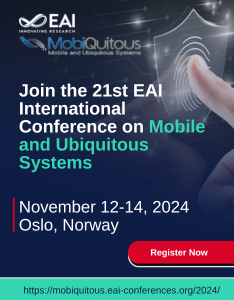
Research Article
Non-sufficient Memories That Are Sufficient for Prediction
547 downloads
@INPROCEEDINGS{10.1007/978-3-642-02466-5_25, author={Wolfgang L\o{}hr and Nihat Ay}, title={Non-sufficient Memories That Are Sufficient for Prediction}, proceedings={Complex Sciences. First International Conference, Complex 2009, Shanghai, China, February 23-25, 2009. Revised Papers, Part 1}, proceedings_a={COMPLEX PART 1}, year={2012}, month={5}, keywords={hidden Markov models HMM computational mechanics causal states -machine prediction}, doi={10.1007/978-3-642-02466-5_25} }
- Wolfgang Löhr
Nihat Ay
Year: 2012
Non-sufficient Memories That Are Sufficient for Prediction
COMPLEX PART 1
Springer
DOI: 10.1007/978-3-642-02466-5_25
Abstract
The causal states of computational mechanics define the minimal sufficient (prescient) memory for a given stationary stochastic process. They induce the -machine which is a hidden Markov model (HMM) generating the process. The -machine is, however, not the minimal generative HMM and minimal internal state entropy of a generative HMM is a tighter upper bound for excess entropy than provided by statistical complexity. We propose a notion of prediction that does not require sufficiency. The corresponding models can be substantially smaller than the -machine and are closely related to generative HMMs.
Copyright © 2009–2024 ICST