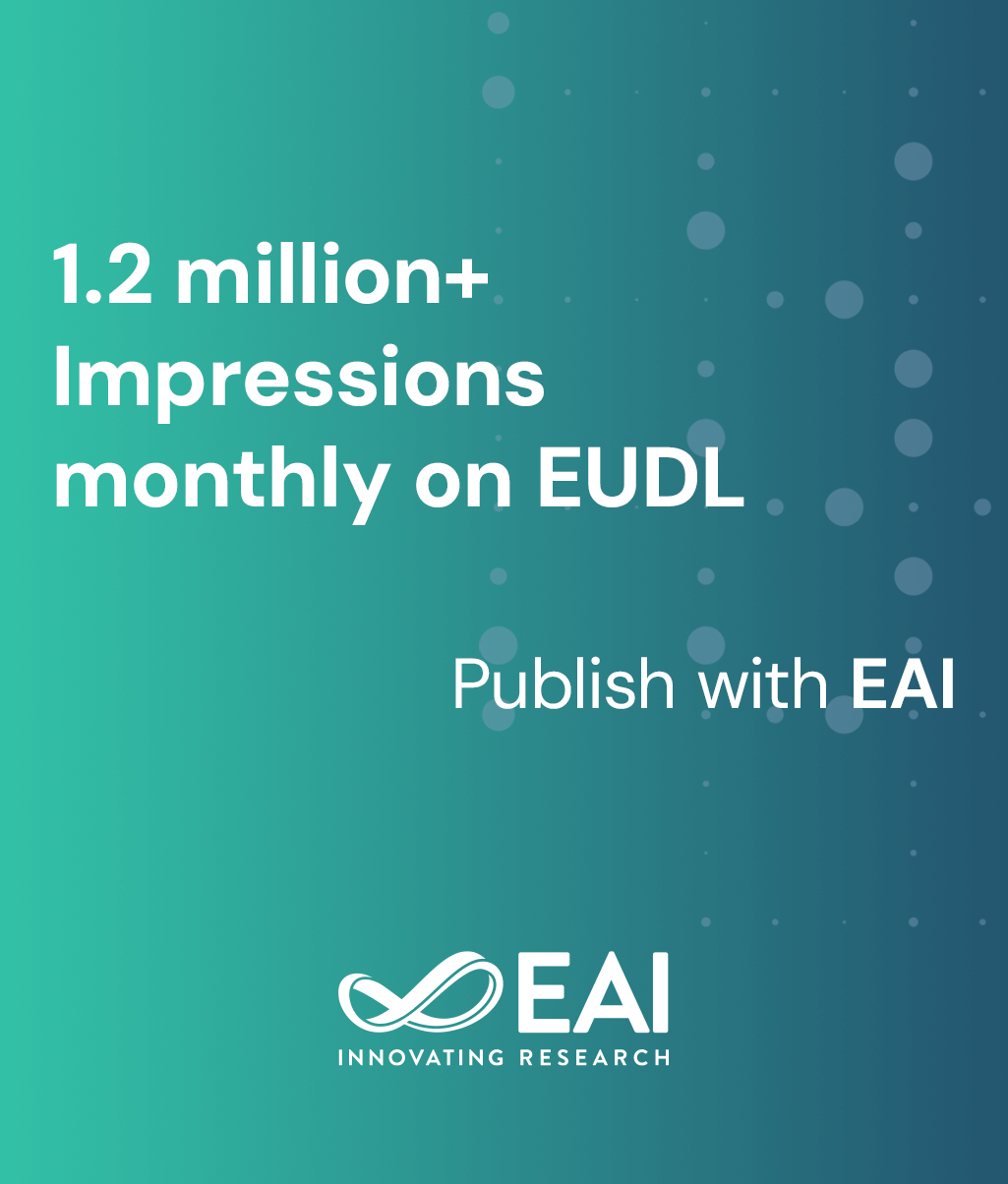
Research Article
Disaster Management in Smart Cities by Forecasting Traffic Plan Using Deep Learning and GPUs
@INPROCEEDINGS{10.1007/978-3-319-94180-6_15, author={Muhammad Aqib and Rashid Mehmood and Aiiad Albeshri and Ahmed Alzahrani}, title={Disaster Management in Smart Cities by Forecasting Traffic Plan Using Deep Learning and GPUs}, proceedings={Smart Societies, Infrastructure, Technologies and Applications. First International Conference, SCITA 2017, Jeddah, Saudi Arabia, November 27--29, 2017, Proceedings}, proceedings_a={SCITA}, year={2018}, month={7}, keywords={Smart cities Disaster management Deep learning GPUs Convolution neural networks}, doi={10.1007/978-3-319-94180-6_15} }
- Muhammad Aqib
Rashid Mehmood
Aiiad Albeshri
Ahmed Alzahrani
Year: 2018
Disaster Management in Smart Cities by Forecasting Traffic Plan Using Deep Learning and GPUs
SCITA
Springer
DOI: 10.1007/978-3-319-94180-6_15
Abstract
The importance of disaster management is evident by the increasing number of natural and manmade disasters such as Irma and Manchester attacks. The estimated cost of the recent Irma hurricane is believed to be more than 80 billion USD; more importantly, more than 40 lives have been lost and thousands were misplaced. Disaster management plays a key role in reducing the human and economic losses. In our earlier work, we have developed a disaster management system that uses VANET, cloud computing, and simulations to devise city evacuation strategies. In this paper, we extend our earlier work by using deep learning to predict urban traffic behavior. Moreover, we use GPUs to deal with compute intensive nature of deep learning algorithms. To the best of our knowledge, we are the first to apply deep learning approach in disaster management. We use real-world open road traffic within a city available through the UK Department for Transport. Our results demonstrate the effectiveness of deep learning approach in disaster management and correct prediction of traffic behavior in emergency situations.