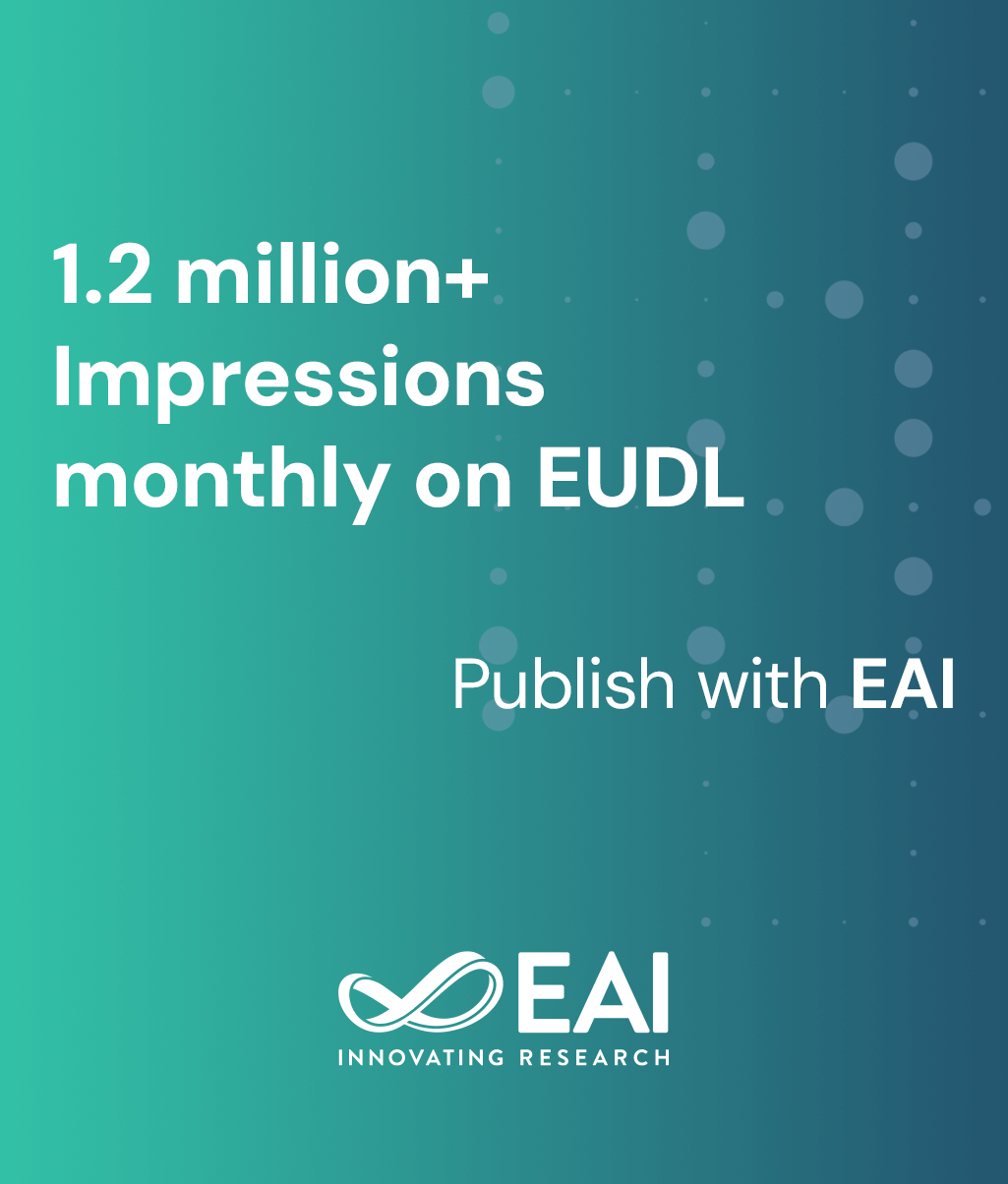
Research Article
Sensor Deployment in Bayesian Compressive Sensing Based Environmental Monitoring
@INPROCEEDINGS{10.1007/978-3-319-11569-6_4, author={Chao Wu and Di Wu and Shulin Yan and Yike Guo}, title={Sensor Deployment in Bayesian Compressive Sensing Based Environmental Monitoring}, proceedings={Mobile and Ubiquitous Systems: Computing, Networking, and Services. 10th International Conference, MOBIQUITOUS 2013, Tokyo, Japan, December 2-4, 2013, Revised Selected Papers}, proceedings_a={MOBIQUITOUS}, year={2014}, month={12}, keywords={Environmental monitoring Compressive sensing Sensor networks Sparse Bayesian learning}, doi={10.1007/978-3-319-11569-6_4} }
- Chao Wu
Di Wu
Shulin Yan
Yike Guo
Year: 2014
Sensor Deployment in Bayesian Compressive Sensing Based Environmental Monitoring
MOBIQUITOUS
Springer
DOI: 10.1007/978-3-319-11569-6_4
Abstract
Sensor networks play crucial roles in the environmental monitoring. So far, the large amount of resource consumption in traditional sensor networks has been a huge challenge for environmental monitoring. Compressive sensing (CS) provides us a method to significantly decrease the number of sensors needed and Bayesian compressive sensing (BCS) makes it possible to deploy sensors selectively rather than randomly. By deploying sensors to the most informative places, we expect to reduce the reconstruction errors further compared with random sensor deployment. In this paper we employ multiple sensor deployment algorithms and BCS based signal recovery algorithm to build novel environmental monitoring systems, in which the environmental signals can be recovered accurately with undersampled measurements. Besides, we apply these environmental monitoring models to ozone data experiments to evaluate them and compare their performance. The results show a significant improvement in the recovery accuracy from random sensor deployment to selective sensor deployment. With 100 measurements for 16641 data points, the reconstruction error of one of the sensor deployment approaches was 40 % less than that of random sensor deployment, with 3.52 % and 6.08 % respectively.