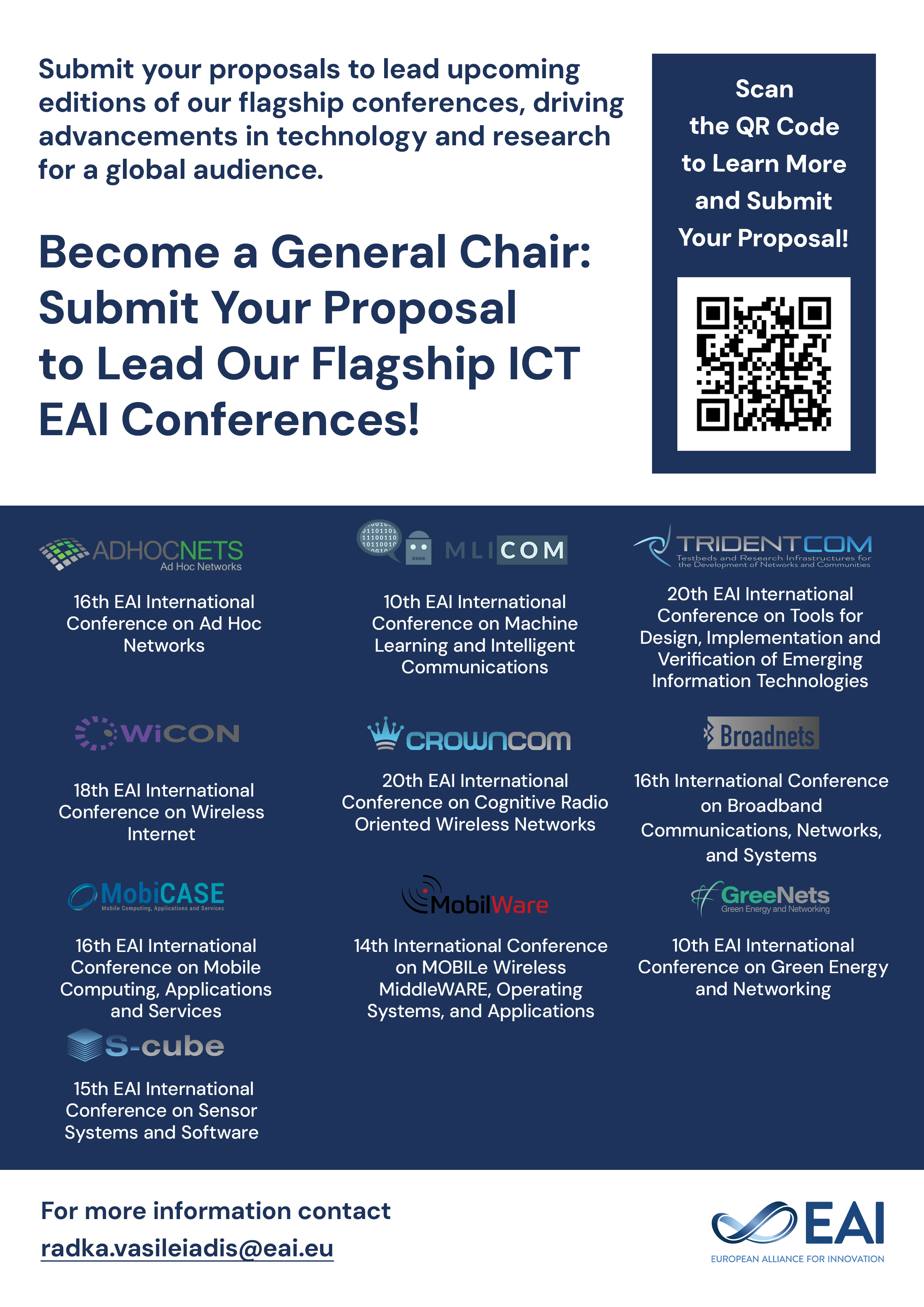
Research Article
Detection of Real-Time Intentions from Micro-blogs
@INPROCEEDINGS{10.1007/978-3-319-11569-6_10, author={Nilanjan Banerjee and Dipanjan Chakraborty and Anupam Joshi and Sumit Mittal and Angshu Rai and B. Ravindran}, title={Detection of Real-Time Intentions from Micro-blogs}, proceedings={Mobile and Ubiquitous Systems: Computing, Networking, and Services. 10th International Conference, MOBIQUITOUS 2013, Tokyo, Japan, December 2-4, 2013, Revised Selected Papers}, proceedings_a={MOBIQUITOUS}, year={2014}, month={12}, keywords={Social networks Micro--blogs Intention mining}, doi={10.1007/978-3-319-11569-6_10} }
- Nilanjan Banerjee
Dipanjan Chakraborty
Anupam Joshi
Sumit Mittal
Angshu Rai
B. Ravindran
Year: 2014
Detection of Real-Time Intentions from Micro-blogs
MOBIQUITOUS
Springer
DOI: 10.1007/978-3-319-11569-6_10
Abstract
Micro-blog forums, such as Twitter, constitute a powerful medium today that people use to express their thoughts and intentions on a daily, and in many cases, hourly, basis. Extracting ‘Real-Time Intention’ (RTI) of a user from such short text updates is a huge opportunity towards web personalization and social networking around dynamic user context. In this paper, we propose novel ensemble approaches for learning and classifying RTI expressions from micro-blogs, based on a wide spectrum of linguistic and statistical features of RTI expressions ( high dimensionality, sparseness of data, limited context, grammatical in-correctness, etc.). We demonstrate our approach achieves significant improvement in accuracy, compared to word-level features used in many social media classification tasks. Further, we conduct experiments to study the run-time performance of such classifiers for integration with a variety of applications. Finally, a prototype implementation using an Android-based user device demonstrates how user context (intention) derived from social media sites can be consumed by novel social networking applications.