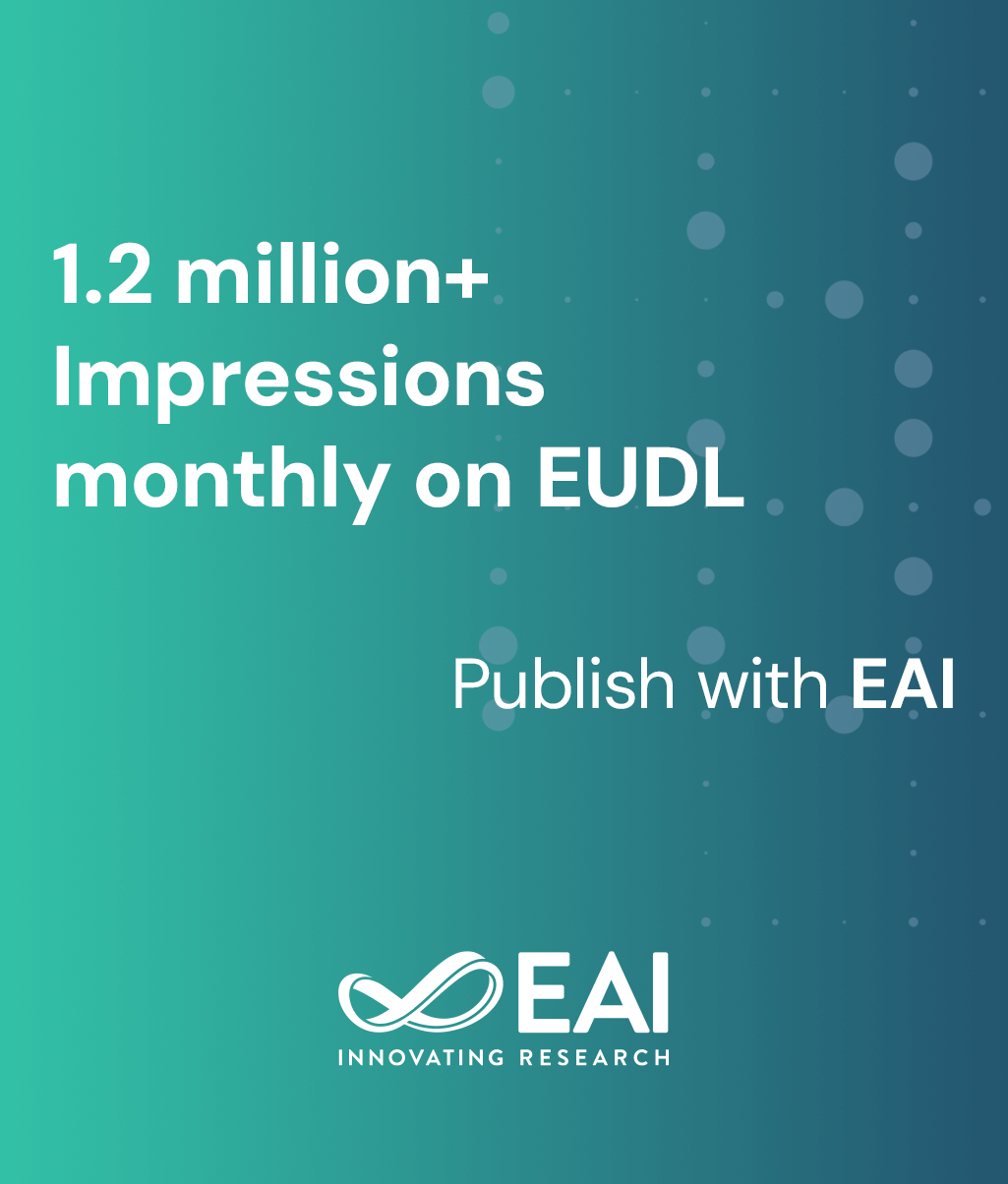
Research Article
MalShoot: Shooting Malicious Domains Through Graph Embedding on Passive DNS Data
@INPROCEEDINGS{10.1007/978-3-030-12981-1_34, author={Chengwei Peng and Xiaochun Yun and Yongzheng Zhang and Shuhao Li}, title={MalShoot: Shooting Malicious Domains Through Graph Embedding on Passive DNS Data}, proceedings={Collaborative Computing: Networking, Applications and Worksharing. 14th EAI International Conference, CollaborateCom 2018, Shanghai, China, December 1-3, 2018, Proceedings}, proceedings_a={COLLABORATECOM}, year={2019}, month={2}, keywords={Domain reputation Graph embedding Domain representation Malicious domains detection}, doi={10.1007/978-3-030-12981-1_34} }
- Chengwei Peng
Xiaochun Yun
Yongzheng Zhang
Shuhao Li
Year: 2019
MalShoot: Shooting Malicious Domains Through Graph Embedding on Passive DNS Data
COLLABORATECOM
Springer
DOI: 10.1007/978-3-030-12981-1_34
Abstract
Malicious domains are key components to a variety of illicit online activities. We propose , a graph embedding technique for detecting malicious domains using passive DNS database. We base its design on the intuition that a group of domains that share similar resolution information would have the same property, namely malicious or benign. represents every domain as a low-dimensional vector according to its DNS resolution information. It automatically maps the domains that share similar resolution information to similar vectors while unrelated domains to distant vectors. Based on the vectorized representation of each domain, a machine-learning classifier is trained over a labeled dataset and is further applied to detect other malicious domains. We evaluate using real-world DNS traffic collected from three ISP networks in China over two months. The experimental results show our approach can effectively detect malicious domains with a 96.08% true positive rate and a 0.1% false positive rate. Moreover, scales well even in large datasets.