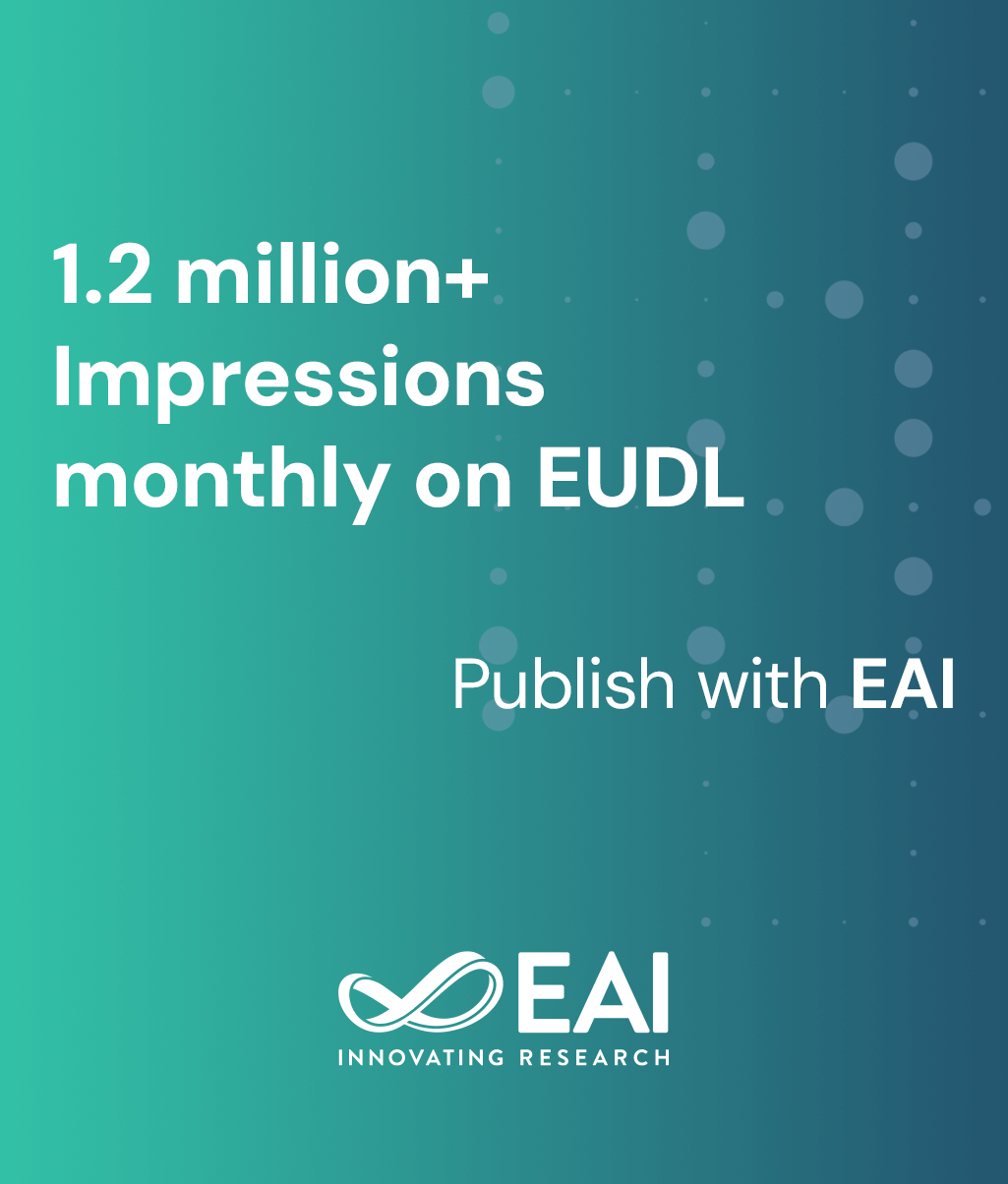
Research Article
RFID Data-Driven Vehicle Speed Prediction Using Adaptive Kalman Filter
@INPROCEEDINGS{10.1007/978-3-030-00557-3_5, author={Anqi Feng and Liping Qian and Yupin Huang}, title={RFID Data-Driven Vehicle Speed Prediction Using Adaptive Kalman Filter}, proceedings={Machine Learning and Intelligent Communications. Third International Conference, MLICOM 2018, Hangzhou, China, July 6-8, 2018, Proceedings}, proceedings_a={MLICOM}, year={2018}, month={10}, keywords={Speed prediction RFID Data acquisition Adaptive Kalman filter}, doi={10.1007/978-3-030-00557-3_5} }
- Anqi Feng
Liping Qian
Yupin Huang
Year: 2018
RFID Data-Driven Vehicle Speed Prediction Using Adaptive Kalman Filter
MLICOM
Springer
DOI: 10.1007/978-3-030-00557-3_5
Abstract
This paper focuses on the design of radio frequency identification (RFID) data-driven vehicle speed prediction method using adaptive Kalman filtering. First of all, when the vehicle moves through a RFID tag, the reader needs to acquire the state information (i.e., current speed and time stamp) of the last vehicle across the tag, and meanwhile transmits its state information to this tag. Then, the state space model can be formulated according to the acquired state information. Finally, the adaptive Kalman filtering algorithm is proposed to predict and adjust the speed of vehicles. Adaptive Kalman filtering algorithm achieves the adaptive updating of variable forgetting factor by analyzing the error between the expected output value and the actual output value, so as to achieve the online updating of the prediction model. The numerical results further show that compared with the conventional Kalman filtering algorithm, the proposed algorithm can increase the speed prediction accuracy by 20%. This implies that the proposed algorithm can provide the better real-time effectiveness for the practical implementation.